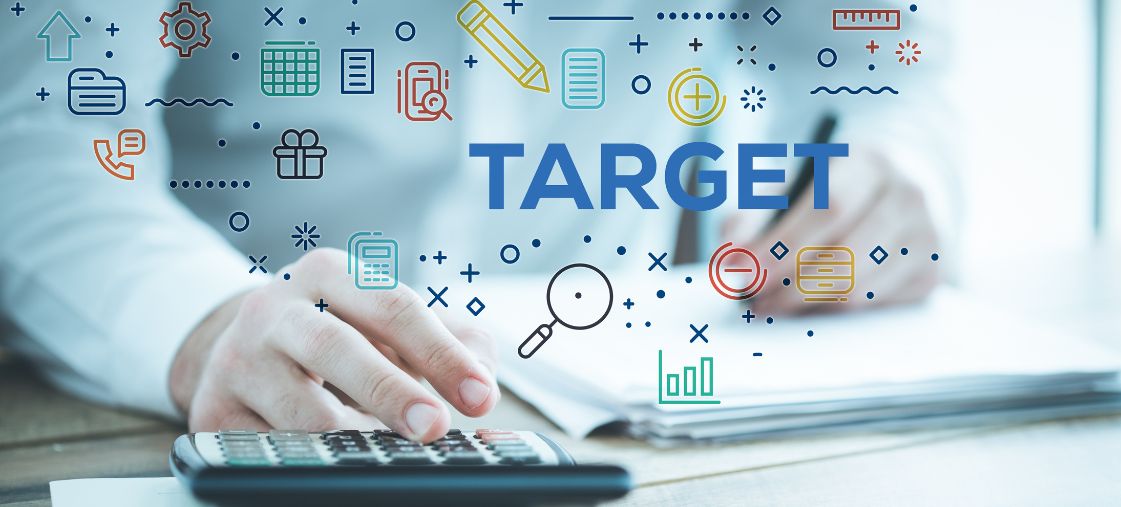
Predictive Analytics for Ad Targeting: Transforming Campaign Performance
Predictive analytics for ad targeting has revolutionized how marketers connect with their audiences, enabling unprecedented precision in campaign delivery and performance. By leveraging advanced algorithms and machine learning models, businesses can now forecast consumer behavior, identify high-value prospects, and deliver personalized advertising experiences that dramatically improve conversion rates. This data-driven approach represents a fundamental shift from traditional demographic targeting to sophisticated behavioral prediction that anticipates customer needs before they even arise.
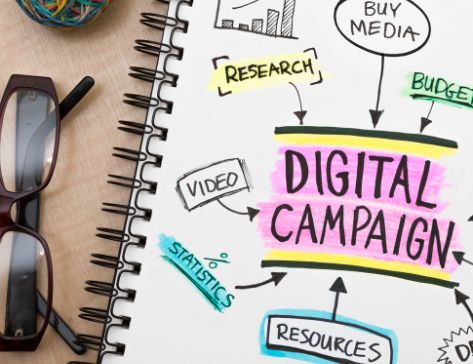
The Evolution of Ad Targeting: From Demographics to Prediction
The journey from basic demographic targeting to predictive analytics represents a quantum leap in advertising effectiveness. Traditional methods relied on broad audience segments based on age, location, and gender—essentially making educated guesses about who might be interested in a product. Modern predictive analytics transforms this approach by analyzing patterns in consumer behavior to forecast future actions with remarkable accuracy.
This evolution has occurred in distinct phases:
Targeting Era | Primary Method | Effectiveness | Data Requirements |
---|---|---|---|
Traditional (Pre-2010) | Demographic segmentation | Low-moderate | Basic customer information |
Behavioral (2010-2015) | Past behavior analysis | Moderate | Interaction history, cookies |
Predictive (2015-2020) | Statistical modeling | High | Multi-source data integration |
AI-Powered (2020-Present) | Machine learning algorithms | Very high | Real-time data streams, unified profiles |
Organizations implementing predictive ad targeting report 30-50% improvements in campaign performance metrics compared to traditional targeting methods. This dramatic enhancement stems from the ability to identify not just who might be interested in an offer, but precisely when they’re most likely to convert.
Core Components of Predictive Analytics for Ad Targeting
1. Data Collection and Integration
Effective predictive targeting begins with comprehensive data collection across multiple touchpoints:
- First-party data: Website interactions, purchase history, app usage
- Second-party data: Partner-shared customer insights
- Third-party data: Market research, demographic information
- Contextual signals: Time, location, device, weather
- Behavioral indicators: Browse patterns, content preferences, engagement metrics
The key challenge lies in unifying these diverse data sources into coherent customer profiles that can feed predictive models. Modern customer data platforms (CDPs) solve this by creating persistent, unified customer profiles that update in real-time as new interactions occur.
2. Predictive Modeling Techniques
Several modeling approaches power predictive ad targeting:
- Regression analysis: Forecasts continuous values like potential spend or lifetime value
- Classification models: Predict categorical outcomes such as “likely to convert” vs. “unlikely to convert”
- Clustering algorithms: Identify natural groupings of customers with similar behavior patterns
- Time series analysis: Detects patterns in sequential data to predict future actions
- Deep learning: Processes complex, unstructured data like images and natural language
The most sophisticated platforms employ ensemble methods that combine multiple models to achieve greater accuracy than any single approach could provide.
3. Real-Time Decision Engines
Predictive insights must be actionable in milliseconds to influence ad delivery decisions. Modern platforms use real-time decision engines that can:
- Process incoming user signals instantaneously
- Apply predictive models to determine optimal targeting parameters
- Select the most effective creative variation
- Adjust bid amounts based on conversion probability
- Deliver personalized ad experiences across channels
Implementation Tip: Start with High-Impact Use Cases
When implementing predictive analytics for ad targeting, begin with use cases that offer clear ROI:
- Churn prediction: Identify customers at risk of leaving and target them with retention offers
- Purchase propensity: Focus ad spend on users most likely to convert in the near term
- Lifetime value prediction: Allocate higher budgets to acquiring customers with high LTV potential
- Next best action: Determine the optimal next offer for each customer segment
These focused applications deliver measurable results while building organizational confidence in predictive approaches.
Implementing Predictive Ad Targeting: A Step-by-Step Framework
Successfully deploying predictive analytics for ad targeting requires a structured approach:
Step 1: Define Clear Objectives and KPIs
Begin by establishing specific goals for your predictive targeting initiative:
- Increase conversion rates by X%
- Reduce customer acquisition costs by Y%
- Improve return on ad spend (ROAS) by Z%
- Enhance customer lifetime value
- Decrease ad fatigue and improve relevance scores
These objectives should align with broader business goals and provide clear metrics for measuring success.
Step 2: Audit Available Data Sources
Conduct a comprehensive inventory of your data assets:
- Identify all first-party data sources (CRM, website, app, sales data)
- Evaluate quality, completeness, and accessibility of each source
- Determine what additional data might be needed
- Assess data privacy compliance requirements
- Establish data governance protocols
Step 3: Develop Unified Customer Profiles
Create a single customer view by:
- Implementing identity resolution across devices and channels
- Consolidating behavioral and transactional data
- Enriching profiles with relevant third-party data
- Ensuring real-time profile updates
- Maintaining compliance with privacy regulations
Step 4: Build and Train Predictive Models
Develop models that address your specific objectives:
- Select appropriate modeling techniques based on use cases
- Prepare training data with proper feature engineering
- Train initial models using historical data
- Validate models using holdout samples
- Implement continuous learning mechanisms
Step 5: Integrate with Ad Platforms
Connect predictive insights to your advertising ecosystem:
- Develop APIs for real-time data exchange
- Create custom audiences based on predictive segments
- Implement dynamic creative optimization
- Configure automated bidding strategies
- Establish cross-channel orchestration
Step 6: Test, Measure, and Refine
Continuously improve your predictive targeting approach:
- Conduct A/B tests comparing predictive vs. traditional targeting
- Monitor model performance and accuracy over time
- Refine models based on new data and outcomes
- Expand to additional use cases as capabilities mature
- Document learnings and best practices
Case Study: E-commerce Retailer Transforms Ad Performance
A mid-sized online retailer implemented predictive analytics for their digital advertising with impressive results:
- Challenge: High ad spend with inconsistent returns across product categories
- Solution: Deployed predictive models to identify high-propensity customers for each product line
- Implementation: Created dynamic audience segments updated daily based on browsing patterns and purchase likelihood
- Results:
- 42% increase in conversion rates
- 31% reduction in cost per acquisition
- 2.8x improvement in ROAS
- 22% increase in average order value
The key insight: Predictive models identified subtle patterns in browsing behavior that strongly correlated with purchase intent, allowing for much more precise ad targeting than traditional methods.
Advanced Techniques in Predictive Ad Targeting
Contextual Intelligence
Modern predictive targeting incorporates contextual factors that significantly influence conversion probability:
- Temporal context: Time of day, day of week, seasonality
- Location intelligence: Geographic position, proximity to stores
- Device context: Mobile vs. desktop, app vs. web
- Environmental factors: Weather conditions, local events
- Content context: Surrounding content themes and sentiment
By incorporating these contextual signals, predictive models can determine not just who to target, but when, where, and how to deliver messages for maximum impact.
Multi-touch Attribution Models
Advanced predictive targeting leverages sophisticated attribution models to understand the complex customer journey:
- Data-driven attribution: Uses machine learning to assign fractional credit to touchpoints
- Markov chain models: Analyzes the probability of conversion paths
- Shapley value attribution: Applies game theory to determine touchpoint contributions
- Survival analysis: Examines time-to-conversion across different paths
These models provide crucial feedback to predictive targeting systems, helping refine future targeting decisions based on actual contribution to conversions.
Predictive Audience Suppression
Sometimes knowing who not to target is as valuable as knowing who to target. Predictive suppression identifies:
- Users with extremely low conversion probability
- Recently converted customers who don’t need the same message
- Individuals likely to purchase regardless of ad exposure
- Users showing signs of ad fatigue or negative sentiment
By suppressing these audiences, marketers can reallocate budget to higher-potential prospects and avoid creating negative brand experiences.
Overcoming Common Challenges in Predictive Ad Targeting
Data Privacy and Compliance
As privacy regulations tighten globally, predictive targeting must adapt:
- Implement privacy-by-design principles in data collection
- Develop consent management frameworks
- Explore privacy-preserving analytics techniques
- Leverage first-party data strategies
- Adopt contextual targeting as a privacy-friendly alternative
Organizations that proactively address privacy concerns can maintain targeting effectiveness while building consumer trust.
Data Quality and Integration Issues
Predictive models are only as good as the data that feeds them:
- Implement data quality monitoring and cleansing processes
- Develop robust identity resolution capabilities
- Create data governance frameworks
- Establish real-time data pipelines
- Validate data accuracy through regular audits
Organizational Readiness
Technical capabilities are only part of the equation:
- Develop cross-functional teams spanning analytics and marketing
- Invest in upskilling marketing teams on data interpretation
- Create clear processes for acting on predictive insights
- Establish executive sponsorship for data-driven initiatives
- Implement change management strategies
Implementation Tip: The Crawl-Walk-Run Approach
Successful organizations typically follow a phased implementation:
- Crawl: Start with basic predictive segments applied to a single channel
- Walk: Expand to multi-channel orchestration with more sophisticated models
- Run: Implement real-time, AI-driven decisioning across the customer journey
This incremental approach builds capabilities while delivering measurable wins at each stage.
The Future of Predictive Analytics in Ad Targeting
Several emerging trends will shape the evolution of predictive targeting in the coming years:
AI-Generated Creative Optimization
Predictive analytics is expanding beyond audience selection to optimize creative elements:
- Automated generation of ad variations based on performance data
- Real-time creative assembly tailored to individual preferences
- Multivariate testing at unprecedented scale
- Emotional response prediction to optimize messaging
This convergence of creative and analytics represents the next frontier in advertising personalization.
Federated Learning and Privacy-Preserving Analytics
As third-party cookies disappear, new approaches are emerging:
- Federated learning that keeps personal data on user devices
- Differential privacy techniques that protect individual identity
- Edge computing for local processing of sensitive data
- Cohort-based targeting that maintains anonymity
These technologies will enable predictive targeting to thrive even in a privacy-first ecosystem.
Cross-Platform Identity Resolution
The future of targeting lies in connecting fragmented customer journeys:
- Probabilistic matching across devices and platforms
- Persistent identity graphs that span online and offline interactions
- Integration of emerging channels like connected TV and voice
- Unified measurement across the entire customer experience
As identity resolution improves, predictive targeting will become increasingly seamless across touchpoints.
Autonomous Marketing Systems
The ultimate evolution is toward self-optimizing marketing systems that:
- Continuously test and learn without human intervention
- Automatically allocate budgets across channels and campaigns
- Identify new audience segments based on emerging patterns
- Adapt to market changes in real-time
- Generate insights and recommendations for strategic decisions
Conclusion: The Predictive Advantage
Predictive analytics has fundamentally transformed ad targeting from an art based on intuition to a science driven by data. By forecasting consumer behavior and preferences with increasing accuracy, marketers can deliver more relevant, timely, and effective advertising experiences that benefit both businesses and consumers.
The organizations that gain competitive advantage in this new landscape will be those that successfully integrate predictive capabilities throughout their marketing stack, creating seamless connections between data, insights, and action. As technologies continue to evolve, the gap between leaders and laggards in predictive ad targeting will only widen, making it an essential capability for forward-thinking marketers.
The future belongs to marketers who can not only collect and analyze data but transform it into predictive intelligence that drives meaningful customer connections and business outcomes. By embracing the frameworks and approaches outlined in this guide, organizations can begin their journey toward more effective, efficient, and customer-centric advertising.
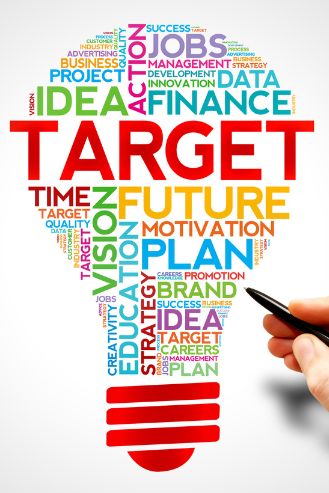