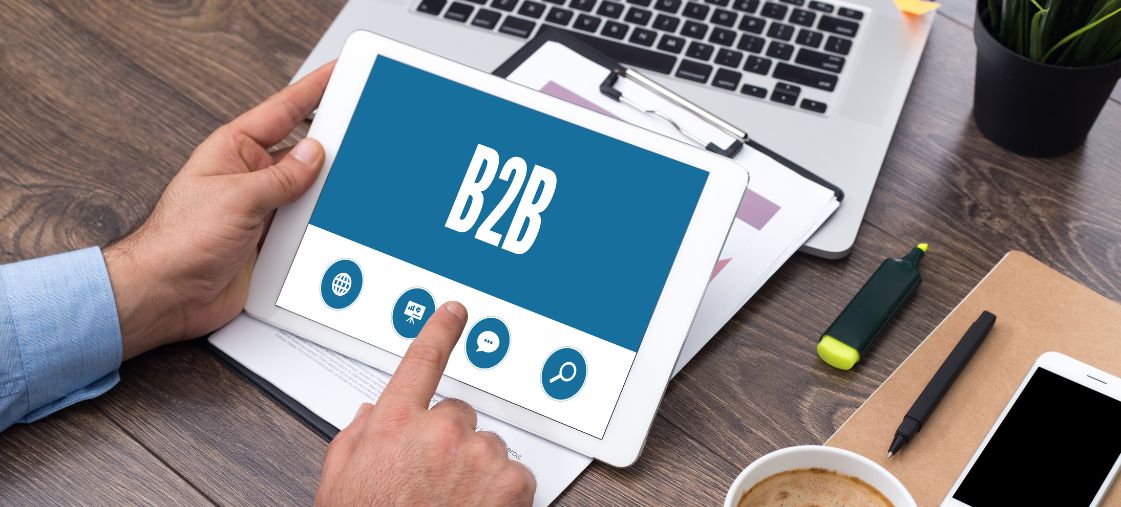
Unlocking Sales Efficiency: The Power of Predictive Lead Scoring in B2B Marketing
In the complex world of business-to-business sales cycles, the effective implementation of Predictive Lead Scoring B2B strategies represents a monumental leap forward, empowering marketing and sales teams to focus their efforts where they matter most. Traditional lead scoring methods, often based on manual rules and limited data points, frequently struggle to accurately identify leads with genuine purchase intent amidst the noise. Predictive lead scoring leverages the power of artificial intelligence (AI) and machine learning (ML) to analyze vast datasets, uncover hidden patterns, and assign scores that reflect a lead’s true likelihood to convert, thereby revolutionizing lead prioritization and driving significant improvements in efficiency and revenue.
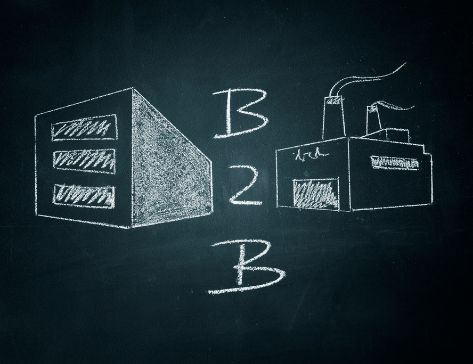
The Shortcomings of Traditional B2B Lead Scoring
For years, B2B marketers have relied on explicit scoring systems. Points are assigned based on demographic data (job title, industry, company size) and behavioral actions (website visits, content downloads, email clicks). While better than no scoring at all, this approach has significant drawbacks. It’s often subjective, relying on marketers’ assumptions about which attributes or actions are most valuable. These rules are static and require constant manual updates to remain relevant. Furthermore, traditional systems struggle to process the complexity and volume of modern customer data or identify subtle correlations between seemingly unrelated actions that might indicate strong buying intent. The effectiveness and impact of various lead scoring models have been studied, highlighting the need for more sophisticated approaches in complex B2B environments.
Enter Predictive Lead Scoring: A Smarter Approach
Predictive lead scoring moves beyond predefined rules. Instead, it uses machine learning algorithms to analyze historical data from both converted and non-converted leads.
The process involves feeding the algorithm data points such as:
- Firmographic Data: Company size, industry, location, revenue, technology stack used.
- Demographic Data: Job title, seniority level, department of individual leads.
- Behavioral Data: Website pages visited, content interactions (downloads, webinar attendance), email engagement, free trial sign-ups, demo requests, social media interactions.
- Engagement Data: Frequency and recency of interactions across multiple channels.
- Historical Sales Data: Information on past deals (won and lost), deal size, sales cycle length.
- Third-Party Intent Data (Optional): Signals indicating active research or interest in relevant topics or competitor solutions from across the web.
The ML model identifies the complex combination of attributes and behaviors that most strongly correlate with successful conversions (e.g., becoming a Marketing Qualified Lead (MQL), Sales Qualified Lead (SQL), or closed-won deal). It then applies this learned model to score new and existing leads based on their resemblance to previously successful leads, providing a dynamic, data-driven probability of conversion.
Why Predictive Lead Scoring is Crucial for B2B Success
The benefits of adopting predictive lead scoring in a B2B context are substantial, impacting alignment, efficiency, and the bottom line. Understanding the nuances of prospect behavior, often revealed through detailed customer journey maps and data analytics, provides the rich input needed for accurate predictive models.
1. Improved Sales and Marketing Alignment
Predictive scoring provides an objective, data-backed definition of a “good lead.” This eliminates subjective debates between sales and marketing about lead quality. Both teams work from the same understanding, fostering trust and collaboration. Marketing focuses on generating leads that fit the predictive model, and sales trusts the scores, knowing they represent genuine potential.
2. Increased Sales Efficiency and Productivity
Sales reps waste less time chasing low-probability leads. By focusing efforts on leads with the highest predictive scores, they can prioritize outreach, tailor their approach more effectively, and engage prospects who are genuinely closer to making a purchase decision. This leads to shorter sales cycles and higher productivity per rep.
3. Higher Conversion Rates and Revenue
By concentrating resources on the most promising leads, businesses naturally see higher conversion rates at each stage of the funnel. More MQLs become SQLs, and more SQLs convert to customers. This directly translates to increased revenue and faster growth.
4. Optimized Marketing Spend and ROI
Marketing teams gain insights into which channels, campaigns, and content pieces generate the highest-scoring leads. This allows them to optimize spend, doubling down on effective tactics and cutting underperforming ones, leading to a significantly better return on marketing investment (ROMI).
5. Deeper Understanding of the Ideal Customer Profile (ICP)
The predictive model implicitly identifies the key characteristics and behaviors of your most valuable customers. Analyzing the factors driving high scores provides data-driven insights into your true ICP, refining targeting and messaging across all marketing efforts.
6. Reduced Lead Fatigue and Improved Nurturing
Leads with lower predictive scores aren’t discarded; they are simply identified as needing more nurturing. Marketing can place these leads into appropriate, automated nurturing tracks designed to educate them and increase their score over time, preventing premature sales outreach and lead fatigue.
How Predictive Lead Scoring Works: A Simplified Overview
- Data Aggregation: Gather historical data on leads (both won and lost) from CRM, Marketing Automation Platform (MAP), website analytics, and potentially third-party sources.
- Define Success: Clearly define the conversion event the model should predict (e.g., MQL status, SQL acceptance, closed-won deal).
- Model Training: Feed the aggregated data into a machine learning algorithm. The algorithm identifies patterns and correlations between lead attributes/behaviors and the defined success event.
- Model Validation: Test the model’s accuracy using a separate dataset to ensure it generalizes well and isn’t just “memorizing” the training data.
- Scoring Deployment: Apply the validated model to incoming and existing leads in real-time or batches. Each lead receives a score indicating their predicted likelihood to convert.
- Integration & Action: Push the predictive scores into the CRM and MAP. Configure workflows to alert sales reps to high-scoring leads, prioritize follow-up queues, and segment leads for targeted nurturing.
- Ongoing Monitoring & Refinement: Continuously monitor model performance and retrain it periodically with new data to ensure it remains accurate as market dynamics and customer behaviors evolve.
Implementing Predictive Lead Scoring: Key Considerations
- Data Quality is Paramount: The model is only as good as the data it’s trained on. Ensure clean, accurate, and sufficient historical data (ideally thousands of records with clear win/loss outcomes).
- Choosing the Right Platform: Many MAPs (like HubSpot, Marketo) offer built-in predictive scoring features. Dedicated AI/predictive analytics platforms provide more customization and power. Evaluate based on needs, budget, and technical resources.
- Sales Team Buy-in: Involve the sales team early. Explain how the scoring works (at a high level) and how it benefits them. Training is crucial for adoption.
- Clear Definition of Success: Be precise about what constitutes a “conversion” for the model to predict.
- Integration Capabilities: Ensure the chosen tool integrates seamlessly with your existing CRM and marketing stack.
- Patience and Iteration: Predictive models take time to build, validate, and refine. It’s an ongoing process, not a one-time setup.
“Focusing on the best leads doesn’t just improve efficiency; it fundamentally changes the quality of sales conversations and accelerates revenue growth.” – B2B Sales Leader Perspective
Conclusion: Embracing the Future of B2B Lead Management
The adoption of Predictive Lead Scoring B2B marks a pivotal shift towards smarter, data-driven sales and marketing operations. By replacing subjective rules with objective, AI-powered predictions, businesses can dramatically improve lead prioritization, enhance sales productivity, boost conversion rates, and achieve superior marketing ROI. It fosters alignment between teams and provides invaluable insights into the ideal customer profile. While implementation requires careful planning and quality data, the competitive advantages gained are undeniable. In an era where efficiency and precision are key, predictive lead scoring isn’t just a nice-to-have; it’s rapidly becoming essential for B2B organizations aiming to optimize their funnel, understand engagement across diverse platforms like those offering native advertising, and ultimately, drive sustainable revenue growth.
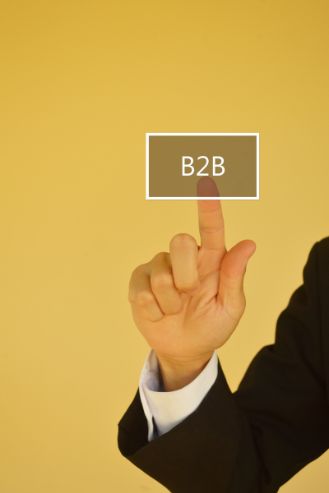