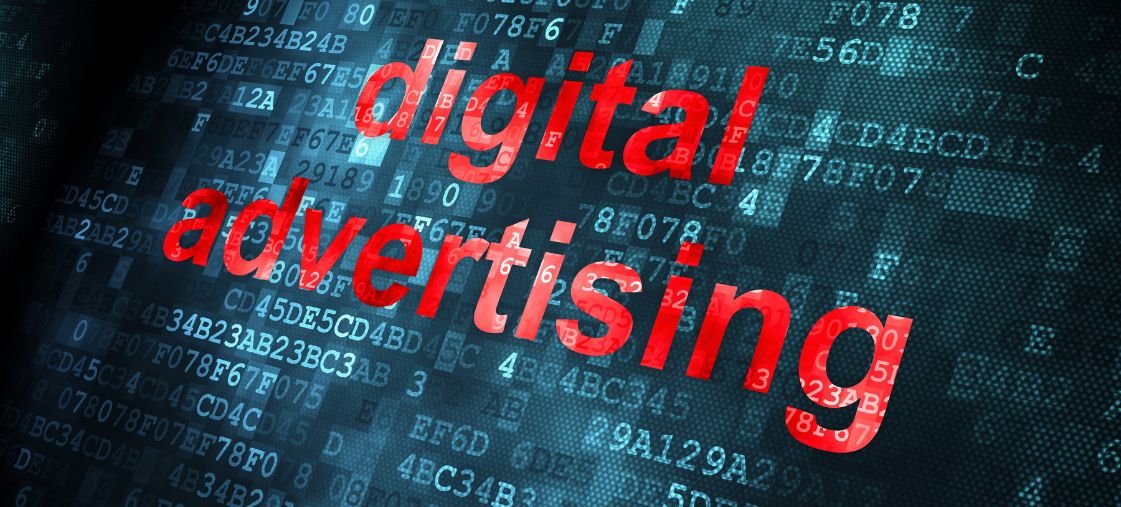
Predictive Marketing: The Next Frontier in Digital Advertising
Representing a paradigm shift from reactive to proactive strategies, Predictive Marketing in Digital Advertising stands as the undisputed next frontier, poised to redefine how brands connect with audiences online. For years, digital advertising relied heavily on analyzing past performance and demographic data to inform future campaigns. While valuable, this approach often resulted in broad targeting, delayed optimization, and missed opportunities. Predictive marketing, powered by artificial intelligence (AI) and machine learning (ML), flips the script by leveraging data to forecast future customer behavior, enabling advertisers to anticipate needs, personalize messages, and optimize campaigns with unprecedented accuracy and efficiency.
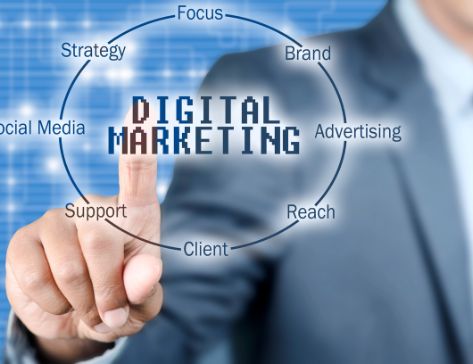
The Limitations of Traditional Digital Advertising
Traditional digital advertising, while revolutionary in its time, operates primarily on hindsight. Campaigns are launched based on historical data, performance is monitored, and adjustments are made reactively. This model faces several inherent limitations in today’s complex digital ecosystem. Targeting often relies on static demographic segments or broad interest categories, which may not capture true purchase intent. Optimization cycles can be slow, meaning budgets might be wasted on underperforming segments or creatives before adjustments are made. Furthermore, it struggles to keep pace with the dynamic, non-linear customer journey and the sheer volume of data generated across multiple touchpoints. While foundational, these methods lack the foresight needed to truly maximize impact in a competitive landscape where anticipating customer needs is paramount, a concept explored further in discussions about predictive analytics as the next frontier.
What is Predictive Marketing? A Shift to Foresight
Predictive marketing utilizes data mining, AI, machine learning, and statistical modeling to make predictions about unknown future events relevant to advertising.
Instead of just asking “What happened?” or “Why did it happen?”, predictive marketing asks “What is likely to happen next?” and “What should we do about it?”. It involves analyzing vast datasets – including historical customer data, real-time behavioral signals, transactional records, and even external factors – to identify patterns and probabilities. Key technologies underpinning predictive marketing include:
- Artificial Intelligence (AI) & Machine Learning (ML): Algorithms that learn from data to identify complex patterns, make predictions (e.g., likelihood to click, convert, or churn), and automate decisions (e.g., ad bidding).
- Big Data Analytics: The ability to process and analyze massive, diverse datasets generated from various sources (web analytics, CRM, social media, ad platforms).
- Customer Data Platforms (CDPs): Technology that creates persistent, unified customer databases accessible to other systems, providing the clean, integrated data needed for accurate modeling.
By harnessing these technologies, predictive marketing enables advertisers to move from reacting to past behavior to proactively engaging audiences based on anticipated future actions.
How Predictive Marketing Transforms Digital Advertising
The application of predictive marketing revolutionizes nearly every facet of digital advertising strategy and execution. It’s not just about incremental improvements; it’s about fundamentally changing how campaigns are planned, targeted, personalized, and optimized. This involves applying many of the principles used to leverage customer data for marketing, but with a specific focus on forecasting future advertising outcomes.
1. Hyper-Targeted Audience Segmentation
Predictive models analyze behavioral, transactional, and demographic data to identify micro-segments with a high propensity to engage or convert. This goes beyond simple interest targeting to find audiences based on predicted future behavior, leading to significantly more relevant ad delivery.
2. Predictive Lead Scoring for Ad Campaigns
For lead generation campaigns, predictive models can score incoming leads based on their likelihood to become paying customers, analyzing data points far beyond basic form fills. This allows advertisers to focus ad spend on acquiring leads predicted to have the highest value.
3. Optimized Ad Spend and Bidding Strategies
Predictive algorithms analyze real-time data to forecast the probability of conversion for each potential ad impression. This powers automated bidding strategies (like Google’s Smart Bidding or Meta’s Advantage+ campaigns) that optimize bids to achieve specific goals (e.g., target CPA, target ROAS) far more effectively than manual bidding.
4. Personalized Ad Creative and Messaging
Predictive insights can inform which ad creatives, messaging angles, or offers are most likely to resonate with specific audience segments or even individual users based on their predicted preferences and stage in the customer journey. This enables dynamic creative optimization (DCO) where ad elements are assembled in real-time for maximum relevance.
5. Churn Prediction for Ad Retargeting
By identifying existing customers or subscribers showing signs of potential churn (based on predicted behavior), advertisers can create highly targeted retargeting campaigns with specific retention offers or win-back messaging, intervening before the customer is lost.
6. Enhanced Lookalike Modeling
Predictive analytics refines lookalike audience creation. Instead of just finding users similar to *all* existing customers, models can identify users similar to those *predicted* to have the highest lifetime value or propensity to purchase specific products, leading to higher-quality prospecting audiences.
7. Dynamic Creative Optimization (DCO) at Scale
Predictive models determine the optimal combination of ad components (headlines, images, calls-to-action, offers) for individual users or micro-segments in real-time, assembling and delivering the most relevant ad variation automatically based on predicted performance.
Implementing Predictive Marketing in Your Digital Advertising Strategy
Adopting predictive marketing requires a strategic approach:
- Define Clear Advertising Objectives: What specific outcomes are you trying to predict and influence (e.g., conversions, lead quality, ROAS, customer retention)? Clear goals guide model development and evaluation.
- Ensure Data Quality and Integration: Predictive models rely heavily on accurate, comprehensive, and integrated data. Invest in data hygiene, break down silos between platforms (CRM, web analytics, ad platforms), and consider a CDP for a unified view.
- Choose the Right Tools and Platforms: Many digital advertising platforms (Google Ads, Meta Ads) have built-in predictive features (automated bidding, dynamic creative). Specialized predictive marketing platforms or AI tools offer more advanced capabilities. Select tools that align with your goals, budget, and technical expertise.
- Develop or Acquire Necessary Skills: Your team needs to understand data analysis principles and how to interpret predictive insights. This might involve training existing staff, hiring data analysts/scientists, or partnering with specialized agencies or consultants.
- Start Small and Test Rigorously: Begin with a specific use case (e.g., implementing predictive bidding on one campaign, testing predictive segmentation for retargeting). Measure results carefully against control groups and iterate based on performance.
- Maintain Ethical Considerations: Be mindful of data privacy regulations (GDPR, CCPA). Ensure transparency in data usage and avoid creating models that perpetuate harmful biases. Focus on providing value to the customer, not just extracting it.
Challenges and the Road Ahead
While transformative, predictive marketing isn’t without challenges:
- Data Privacy Concerns: Navigating evolving regulations and maintaining consumer trust is paramount.
- Model Accuracy and Explainability: Ensuring models are accurate and understanding *why* they make certain predictions (explainability) can be complex.
- Need for Expertise: Building and managing sophisticated predictive models often requires specialized skills.
- Integration Complexity: Integrating predictive insights seamlessly into existing ad workflows and platforms can be technically challenging.
- Cost of Technology: Advanced predictive marketing platforms can represent a significant investment.
Despite these hurdles, the trend is clear. AI and ML capabilities are becoming more accessible, integrated into standard advertising platforms, and crucial for competitive performance. The future likely holds even more sophisticated real-time prediction, deeper personalization, and seamless cross-channel optimization powered by predictive intelligence.
“The best way to predict the future is to create it.” – Peter Drucker. Predictive marketing empowers advertisers to actively shape future outcomes rather than just reacting to past events.
Conclusion: Embracing the Predictive Advertising Era
Predictive Marketing in Digital Advertising represents a fundamental evolution, moving the industry from educated guesses based on the past to informed strategies based on future probabilities. By leveraging AI and machine learning to anticipate customer behavior, advertisers can achieve unprecedented levels of targeting accuracy, personalization, and campaign efficiency. The ability to optimize spend, personalize creative, score leads predictively, and retain customers proactively offers a significant competitive advantage. While implementation requires strategic planning, investment in data and technology, and a commitment to ethical practices, the potential rewards are immense. As predictive capabilities become increasingly integrated into advertising platforms, including those managing diverse formats like native advertising, embracing this next frontier is essential for any brand seeking to maximize its digital advertising impact and thrive in the future.
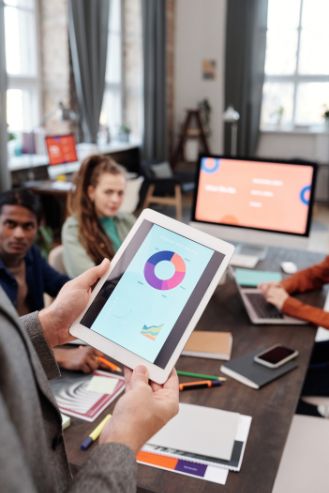