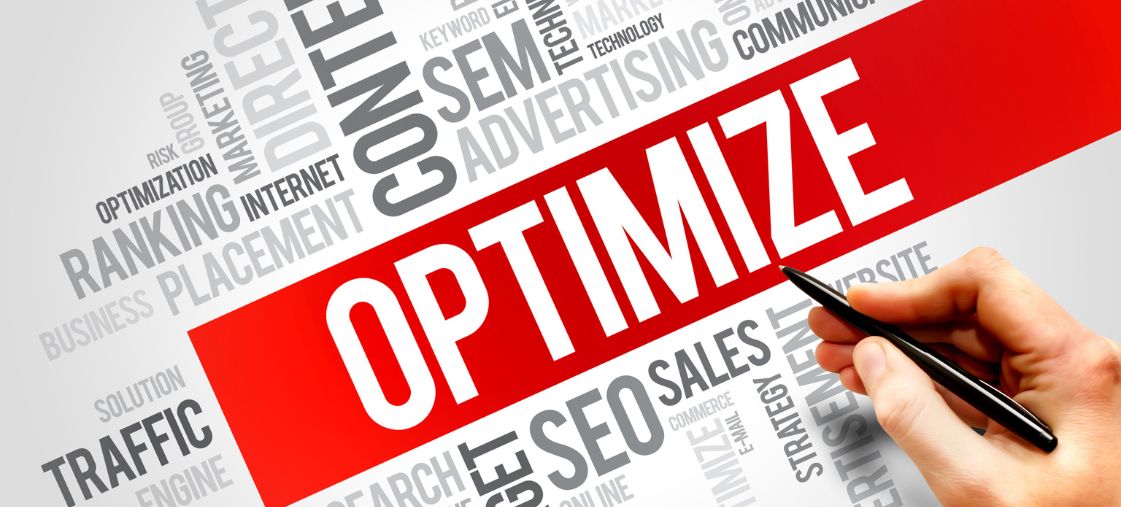
AI Marketing Attribution: Revolutionizing Campaign Measurement and Optimization
AI marketing attribution has fundamentally transformed how businesses measure, analyze, and optimize their marketing efforts across increasingly complex customer journeys. Unlike traditional attribution models that rely on simplified rules and assumptions, AI-powered attribution leverages machine learning algorithms to process vast amounts of cross-channel data, identify meaningful patterns, and deliver more accurate insights into which marketing touchpoints truly influence conversions. This comprehensive guide explores how artificial intelligence is revolutionizing marketing attribution and provides actionable strategies for implementing these advanced approaches in your organization.
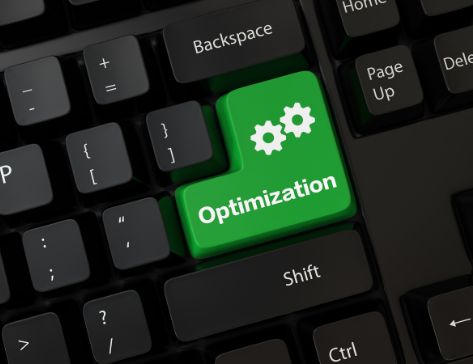
The Evolution of Marketing Attribution
Marketing attribution has evolved dramatically over the past decade, moving from simplistic single-touch models to increasingly sophisticated approaches. This evolution reflects marketers’ ongoing quest to accurately prove their unique value in driving business results. Understanding this progression provides important context for appreciating the transformative impact of AI:
First-Generation Attribution: Single-Touch Models
- First-Touch Attribution: Assigns 100% credit to the first interaction
- Last-Touch Attribution: Assigns 100% credit to the final interaction before conversion
- Limitations: Ignores the complexity of the customer journey and the influence of multiple touchpoints
Second-Generation Attribution: Multi-Touch Models
- Linear Attribution: Distributes credit equally across all touchpoints
- Time-Decay Attribution: Assigns more credit to touchpoints closer to conversion
- Position-Based Attribution: Gives more weight to first and last interactions
- Limitations: Uses predetermined rules rather than data-driven insights to assign credit
Third-Generation Attribution: Algorithmic Models
- Statistical Modeling: Uses regression analysis to determine touchpoint influence
- Markov Chain Models: Analyzes the probability of moving from one touchpoint to another
- Limitations: Requires significant data science expertise and often lacks real-time capabilities
Fourth-Generation Attribution: AI-Powered Models
- Machine Learning Attribution: Continuously learns from data patterns to improve accuracy
- Deep Learning Models: Processes complex, unstructured data across channels
- Advantages: Delivers dynamic, personalized attribution that adapts to changing conditions
Organizations using AI-powered attribution models report an average 20-30% improvement in marketing ROI compared to those using traditional attribution approaches, according to recent industry research.
How AI Transforms Marketing Attribution
Artificial intelligence brings several revolutionary capabilities to marketing attribution:
1. Processing Massive, Cross-Channel Data Sets
AI systems can ingest and analyze enormous volumes of data from diverse sources—including web analytics, CRM, advertising platforms, offline channels, and customer service interactions—creating a comprehensive view of the customer journey that would be impossible to achieve manually.
2. Identifying Non-Linear Relationships
Machine learning algorithms excel at discovering complex, non-linear relationships between marketing activities and outcomes, revealing how touchpoints interact with and influence each other in ways that rule-based models cannot detect.
3. Accounting for External Factors
AI attribution can incorporate external variables such as seasonality, competitor activities, economic conditions, and even weather patterns to provide context for marketing performance and isolate the true impact of your campaigns.
4. Delivering Individualized Attribution
Rather than applying a one-size-fits-all model, AI can create personalized attribution models for different customer segments, products, or markets, recognizing that the path to purchase varies significantly across these dimensions.
5. Providing Predictive Insights
Beyond explaining past performance, AI attribution can forecast future outcomes, enabling marketers to simulate the impact of different budget allocations and campaign strategies before committing resources.
6. Continuous Learning and Adaptation
Unlike static models, AI attribution systems continuously learn from new data, automatically adapting to changing customer behaviors, market conditions, and marketing tactics without requiring manual recalibration.
Key AI Attribution Models and Approaches
Probabilistic Attribution
How it works: Uses Bayesian networks and probabilistic modeling to determine the likelihood that specific touchpoints influenced conversion.
Best for: Organizations with moderate data volume but complex customer journeys.
Advantages: Handles uncertainty well, works with incomplete data, provides confidence intervals.
Machine Learning Attribution
How it works: Employs supervised learning algorithms (random forests, gradient boosting) to identify patterns in historical conversion data.
Best for: Companies with substantial historical data and diverse marketing channels.
Advantages: Handles large feature sets, identifies non-linear relationships, relatively interpretable.
Deep Learning Attribution
How it works: Utilizes neural networks to process vast amounts of structured and unstructured data across the customer journey.
Best for: Enterprises with massive data sets including diverse data types (text, images, video).
Advantages: Highest potential accuracy, can incorporate content data, handles complex patterns.
Reinforcement Learning Attribution
How it works: Learns optimal attribution by testing different models and improving based on actual business outcomes.
Best for: Organizations focused on continuous optimization and with ability to experiment.
Advantages: Self-improving over time, directly tied to business results, adapts to changing conditions.
Implementing AI Attribution: A Step-by-Step Framework
Follow this systematic approach to successfully implement AI-powered attribution in your organization:
Step 1: Assess Your Attribution Readiness
Begin by evaluating your current capabilities and data infrastructure:
- Audit existing data sources and quality
- Evaluate tracking implementation across channels
- Identify gaps in customer journey visibility
- Assess analytical capabilities and resources
- Review current attribution approaches and limitations
Output: Attribution readiness assessment with prioritized improvement areas
Step 2: Define Clear Business Objectives
Clarify what you want to achieve with improved attribution:
- Identify specific business questions to answer
- Define key performance indicators (KPIs) to optimize
- Establish success criteria for the attribution initiative
- Determine how attribution insights will inform decisions
- Secure stakeholder alignment on objectives
Output: Attribution charter with clear goals and success metrics
Step 3: Implement Comprehensive Tracking
Ensure you’re capturing all relevant customer interactions:
- Deploy consistent tracking across digital touchpoints
- Integrate offline channel data where possible
- Implement cross-device and cross-channel identity resolution
- Capture contextual data beyond simple interactions
- Ensure privacy compliance in all data collection
Output: Comprehensive tracking implementation that provides a complete view of the customer journey
Step 4: Select and Implement the Right AI Attribution Approach
Choose the appropriate model based on your needs and capabilities:
- Evaluate different AI attribution technologies and vendors
- Consider build vs. buy decisions based on internal capabilities
- Start with a focused scope before expanding
- Implement proper integration with existing marketing systems
- Establish baseline measurements for comparison
Output: Implemented AI attribution solution integrated with marketing ecosystem
Step 5: Validate and Calibrate the Model
Ensure your attribution model delivers accurate, actionable insights:
- Compare model outputs against controlled experiments
- Conduct incremental lift testing to validate findings
- Gather feedback from marketing teams on insight quality
- Make necessary adjustments to improve accuracy
- Document model assumptions and limitations
Output: Validated attribution model with documented accuracy and confidence levels
Step 6: Operationalize Attribution Insights
Integrate attribution intelligence into marketing workflows:
- Develop actionable dashboards and reporting
- Create processes for regular budget reallocation
- Implement automated optimization where possible
- Train marketing teams on using attribution insights
- Establish feedback loops to continuously improve the model
Output: Operational framework for translating attribution insights into marketing actions
Pro Tip: When implementing AI attribution, start with a hybrid approach that runs your new AI model alongside your existing attribution method. This parallel implementation allows you to compare outputs, build confidence in the new approach, and ensure a smooth transition without disrupting ongoing marketing operations.
Overcoming Common AI Attribution Challenges
Address these typical obstacles to successfully implement AI-powered attribution:
Data Quality and Integration Issues
Solution: Implement data governance protocols, establish clear data definitions, and invest in customer data platforms (CDPs) that create unified customer profiles across touchpoints. Prioritize data quality over quantity initially.
Privacy Regulations and Cookie Deprecation
Solution: Develop a first-party data strategy, implement privacy-preserving measurement approaches like data clean rooms, and explore modeled conversions and probabilistic matching techniques that comply with regulations.
Organizational Resistance
Solution: Start with pilot projects that demonstrate clear ROI, involve stakeholders early in the process, and focus on how attribution insights can help teams achieve their goals rather than threatening existing practices.
Attribution Model Transparency
Solution: Select AI approaches that provide explainable outputs, supplement model insights with controlled experiments, and develop intuitive visualizations that help stakeholders understand key findings.
Technical Complexity
Solution: Consider attribution-as-a-service solutions that reduce implementation burden, partner with specialized analytics providers, and build internal capabilities incrementally through training and focused hiring.
Case Study: Financial Services Company
A leading financial services firm implemented an AI-powered attribution solution to optimize their complex marketing mix spanning digital advertising, content marketing, email nurturing, and offline channels. The company had previously relied on last-click attribution, which significantly undervalued their content marketing and early-funnel activities.
Implementation approach:
- Deployed a machine learning attribution model that incorporated both online and offline touchpoints
- Integrated contextual data including competitor activity and economic indicators
- Created segment-specific attribution models for different product lines
- Implemented a continuous testing framework to validate attribution findings
Results:
- Identified that content marketing was driving 3x more conversions than previously recognized
- Discovered specific channel synergies that increased conversion rates by 45% when activated together
- Reduced customer acquisition costs by 28% through optimized channel allocation
- Improved marketing forecast accuracy by 40%
Future Trends in AI Marketing Attribution
Stay ahead of the curve by monitoring these emerging developments:
- Cookieless Attribution: New methodologies that maintain measurement accuracy in a world without third-party cookies
- Unified Marketing Measurement: Approaches that combine attribution with marketing mix modeling for comprehensive insights
- Real-Time Attribution: Systems that provide instantaneous feedback for immediate optimization
- Attention-Based Attribution: Models that incorporate actual attention metrics rather than simple exposure or clicks
- Cross-Platform Identity Graphs: Solutions that maintain customer journey visibility across walled gardens
- Causal AI: Advanced techniques that move beyond correlation to establish true causality in marketing impact
Conclusion
AI marketing attribution represents a quantum leap forward in marketers’ ability to understand campaign performance, optimize resource allocation, and demonstrate true business impact. By moving beyond simplistic rules-based approaches to sophisticated machine learning models, organizations can gain unprecedented insight into how their marketing activities collectively influence customer decisions across increasingly complex journeys.
While implementing AI attribution requires investment in data infrastructure, analytical capabilities, and organizational change management, the returns—more efficient marketing spend, improved customer experiences, and stronger business results—make it well worth the effort. As competition intensifies and customer journeys become even more fragmented, AI-powered attribution will become not just a competitive advantage but an essential component of marketing success.
By following the structured implementation approach outlined in this guide and addressing common challenges proactively, marketers can harness the power of artificial intelligence to transform their measurement frameworks and decision-making processes. The future of marketing belongs to organizations that can not only execute compelling campaigns but accurately understand their impact through advanced marketing tools and methodologies that leverage the full potential of AI.
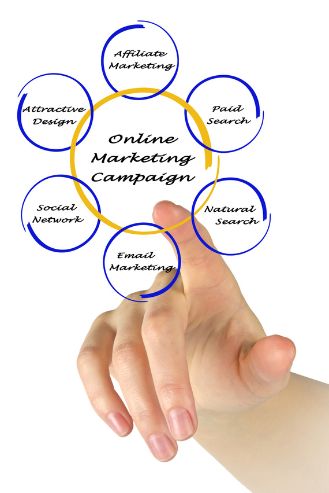