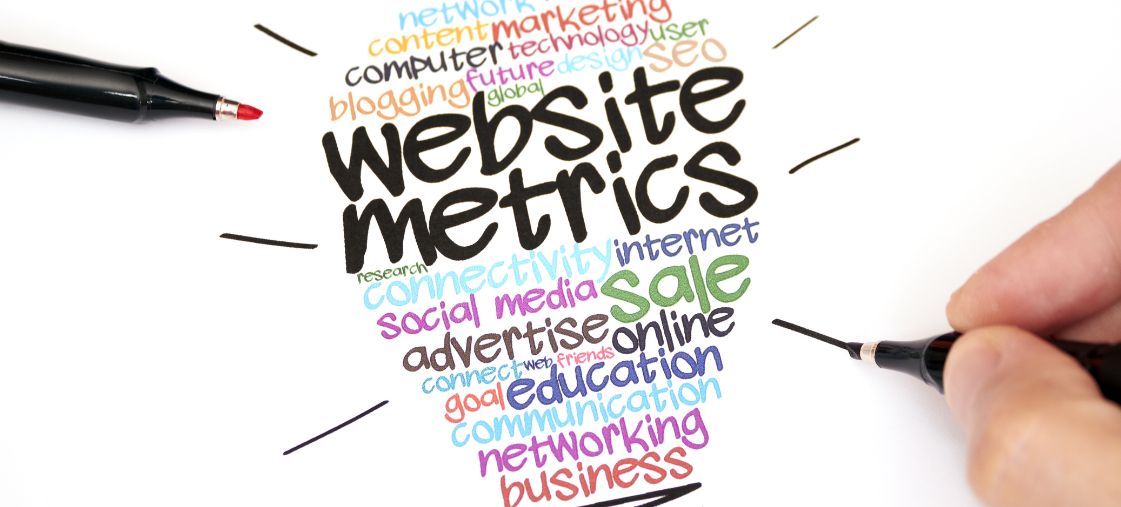
Essential Predictive Marketing Metrics That Drive Business Growth
Predictive marketing metrics have transformed how forward-thinking businesses measure success and plan future campaigns, enabling marketers to shift from reactive analysis to proactive strategy development. Unlike traditional marketing measurements that tell you what happened in the past, predictive metrics leverage advanced analytics and machine learning to forecast future outcomes, identify emerging opportunities, and optimize marketing investments before resources are committed. This comprehensive guide explores the most valuable predictive metrics and how to implement them effectively in your marketing strategy.
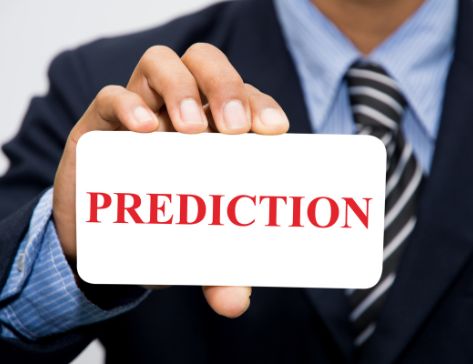
Why Traditional Marketing Metrics Fall Short
While conventional metrics like click-through rates, conversion rates, and cost per acquisition provide valuable historical insights, they offer limited guidance for future decision-making. Modern marketing requires a more forward-looking approach that anticipates customer behaviors, market trends, and campaign performance. Predictive metrics fill this gap by transforming historical data into actionable forecasts that drive more effective marketing strategies.
The limitations of traditional metrics include:
- Backward-looking perspective that doesn’t account for changing conditions
- Inability to identify emerging opportunities before competitors
- Limited guidance for optimal resource allocation
- Reactive rather than proactive approach to marketing challenges
- Difficulty connecting marketing activities to long-term business outcomes
Organizations that prioritize predictive marketing metrics report an average 20-30% improvement in marketing ROI compared to those relying solely on traditional measurements, according to recent industry research.
Core Predictive Marketing Metrics Every Business Should Track
These essential predictive metrics provide forward-looking insights that drive more effective marketing decisions:
1. Customer Lifetime Value Prediction (pCLV)
This metric forecasts the total revenue a customer will generate throughout their relationship with your business, enabling more accurate customer acquisition investment decisions and personalized retention strategies.
Why it matters: pCLV helps marketers identify high-value customer segments, optimize acquisition spending, and develop targeted retention programs that maximize long-term profitability.
How to calculate:
Advanced approach: Implement machine learning models that incorporate behavioral signals, engagement patterns, and demographic factors to create individualized CLV predictions for each customer.
2. Churn Probability Score
This predictive metric assigns each customer a score representing their likelihood of discontinuing their relationship with your business within a specific timeframe, enabling proactive retention efforts.
Why it matters: Identifying at-risk customers before they leave allows for targeted intervention, potentially saving valuable relationships and associated revenue.
How to implement:
- Analyze historical churn patterns to identify leading indicators
- Develop a predictive model using factors like engagement decline, support interactions, and competitive activity
- Assign probability scores to current customers
- Create tiered intervention strategies based on risk level
3. Customer Propensity Models
These models predict the likelihood of specific customer actions, such as making a purchase, upgrading a service, responding to a particular offer, or engaging with certain content types.
Why it matters: Propensity modeling enables highly targeted marketing that delivers the right message to the right customer at the right time, significantly improving conversion rates and marketing efficiency.
Key propensity scores to track:
- Propensity to Purchase: Likelihood of buying in the near future
- Propensity to Upgrade: Probability of moving to a higher-tier product or service
- Propensity to Engage: Likelihood of interacting with specific content or channels
- Propensity to Advocate: Probability of recommending your brand to others
4. Predictive Lead Scoring
This metric assigns values to leads based on their predicted likelihood to convert into customers, helping sales teams prioritize their efforts on the most promising prospects.
Why it matters: Predictive lead scoring dramatically improves sales efficiency by focusing resources on leads with the highest conversion potential, leveraging data science to identify patterns that human analysis might miss.
Implementation approach:
- Analyze historical conversion data to identify predictive factors
- Develop a scoring model that weighs various attributes and behaviors
- Integrate the model with your CRM and marketing automation systems
- Establish score thresholds for different sales actions
- Continuously refine the model based on new conversion data
5. Campaign Lift Prediction
This metric forecasts the incremental impact of marketing campaigns on key performance indicators like revenue, conversion rates, or customer acquisition before campaign launch.
Why it matters: Lift prediction enables marketers to test campaign concepts virtually, optimize messaging and targeting before spending resources, and set realistic performance expectations.
How to implement:
- Analyze historical campaign performance data
- Identify factors that correlate with successful outcomes
- Develop models that predict performance based on campaign attributes
- Use A/B testing data to refine prediction accuracy
- Compare actual results to predictions to improve future forecasts
6. Customer Journey Stage Prediction
This metric forecasts where customers are in their buying journey and when they’re likely to move to the next stage, enabling more relevant messaging and timely interventions.
Why it matters: Understanding a customer’s current journey stage and predicting their progression allows marketers to deliver stage-appropriate content and offers that accelerate the path to purchase.
Key journey stages to predict:
- Awareness to Consideration transition probability
- Consideration to Evaluation timeframe
- Evaluation to Purchase likelihood
- Post-purchase engagement patterns
7. Marketing Mix Optimization Models
These predictive models forecast how different allocations of your marketing budget across channels and tactics will impact overall performance, enabling optimal resource distribution.
Why it matters: Marketing mix modeling helps maximize ROI by directing investments to the channels and activities predicted to deliver the strongest results for specific business objectives.
Implementation approach:
- Gather comprehensive historical data on marketing investments and outcomes
- Account for external factors like seasonality and competitive activity
- Develop models that quantify the impact of each marketing element
- Create scenario planning tools to test different allocation strategies
- Implement continuous measurement and refinement processes
Implementing Predictive Metrics in Your Marketing Strategy
Follow this step-by-step approach to successfully integrate predictive metrics into your marketing operations:
Step 1: Assess Your Data Foundation
Before implementing predictive metrics, evaluate your current data ecosystem:
- Audit available data sources and quality
- Identify gaps in data collection
- Ensure proper data integration across systems
- Establish data governance protocols
- Implement necessary tracking and tagging
Pro Tip: Start with the data you have rather than waiting for perfect information. Begin with simpler predictive models based on available data, then expand as your data collection capabilities improve.
Step 2: Prioritize Metrics Based on Business Impact
Not all predictive metrics will deliver equal value for your specific business. Prioritize based on:
- Alignment with key business objectives
- Potential financial impact
- Implementation complexity and resource requirements
- Data availability and quality for each metric
- Organizational readiness to act on the insights
Step 3: Select Appropriate Analytical Approaches
Different predictive metrics require different analytical techniques:
Metric Type | Recommended Analytical Approaches |
---|---|
Customer-level predictions (CLV, churn) | Regression models, survival analysis, random forests |
Propensity modeling | Logistic regression, gradient boosting, neural networks |
Lead scoring | Classification algorithms, ensemble methods |
Campaign performance | Time series analysis, Bayesian methods |
Marketing mix optimization | Econometric modeling, attribution models |
Step 4: Develop Implementation Roadmap
Create a phased approach to implementing predictive metrics:
- Start with high-impact, lower-complexity metrics
- Establish clear success criteria for each implementation
- Define roles and responsibilities across teams
- Create a timeline with specific milestones
- Identify necessary technology investments
Step 5: Integrate with Marketing Workflows
Ensure predictive insights are actionable by integrating them into daily marketing operations:
- Incorporate predictive metrics into dashboards and reporting
- Establish alert systems for significant prediction changes
- Create standard operating procedures for acting on insights
- Train marketing teams on interpreting and using predictive data
- Align incentives and KPIs with predictive metrics
Step 6: Continuously Evaluate and Refine
Implement processes to maintain and improve your predictive capabilities:
- Regularly compare predictions against actual outcomes
- Monitor model performance and address degradation
- Update models with new data and variables
- Test alternative modeling approaches
- Expand to additional predictive metrics as capabilities mature
Common Challenges and Solutions
Organizations often face these obstacles when implementing predictive marketing metrics:
Data Quality and Integration Issues
Solution: Implement data quality monitoring processes, establish clear data definitions, and invest in integration technologies that create a unified customer view across systems.
Skill Gaps and Technical Complexity
Solution: Consider a hybrid approach that combines internal capability building with external expertise. Look for user-friendly predictive analytics platforms designed for marketers rather than data scientists.
Organizational Resistance
Solution: Start with pilot projects that demonstrate clear ROI, involve stakeholders early in the process, and focus on translating technical insights into business-friendly language and actionable recommendations.
Balancing Complexity and Usability
Solution: Prioritize interpretability alongside accuracy in your predictive models. The most sophisticated model is worthless if marketers don’t understand or trust the insights it generates.
Case Study: A B2B software company implemented predictive lead scoring and customer propensity models, resulting in a 42% increase in sales team efficiency and a 28% improvement in campaign conversion rates within six months. The key to their success was close collaboration between marketing, sales, and data science teams throughout the implementation process.
Future Trends in Predictive Marketing Metrics
Stay ahead of the curve by monitoring these emerging developments:
- AI-Powered Creative Optimization: Predictive metrics that forecast the performance of different creative elements and automatically optimize content
- Real-Time Personalization Metrics: Instantaneous predictions that enable dynamic content adaptation during customer interactions
- Unified Cross-Channel Attribution: Advanced models that accurately predict the impact of marketing activities across increasingly complex customer journeys
- Emotion and Sentiment Prediction: Metrics that forecast emotional responses to marketing messages before campaign launch
- Privacy-Preserving Predictive Analytics: Techniques that maintain predictive power while respecting evolving privacy regulations and consumer preferences
Conclusion
Predictive marketing metrics represent a fundamental shift in how businesses measure and optimize their marketing efforts. By moving beyond retrospective analysis to forward-looking insights, these metrics enable marketers to anticipate customer needs, optimize resource allocation, and maximize return on marketing investments. The result is not just improved campaign performance but a more strategic approach to marketing that drives sustainable business growth.
While implementing predictive metrics requires investment in data infrastructure, analytical capabilities, and marketing tools, the returns—more efficient marketing spend, improved customer experiences, and stronger business results—make it well worth the effort. As competition intensifies and customer expectations continue to rise, predictive marketing metrics will become not just a competitive advantage but an essential component of marketing success.
By following the structured implementation approach outlined in this guide and addressing common challenges proactively, marketers can harness the power of predictive analytics to transform their measurement frameworks and decision-making processes. The future of marketing belongs to organizations that can not only understand what happened yesterday but accurately predict and shape what will happen tomorrow.
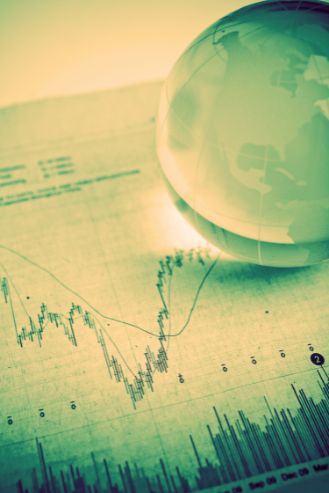