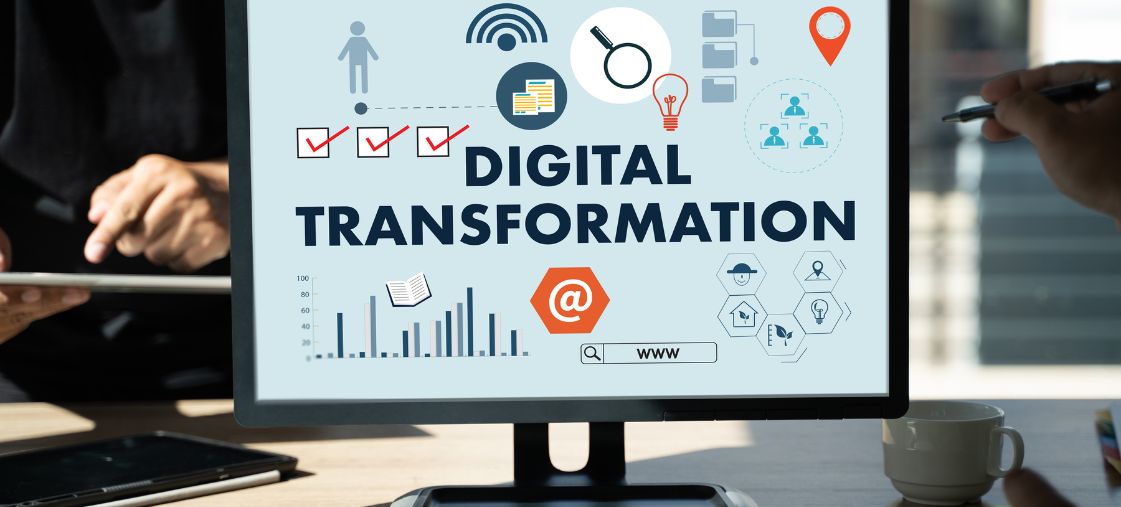
Marketing Data Science: Transforming Strategies in the Digital Age
Marketing data science has revolutionized how brands connect with consumers, turning vast amounts of information into actionable insights that drive more effective campaigns and business growth. This powerful discipline combines statistical analysis, machine learning, and domain expertise to extract meaningful patterns from customer data, enabling marketers to make evidence-based decisions rather than relying on intuition alone. As digital touchpoints multiply and consumer expectations rise, data science has become the cornerstone of modern marketing strategy.
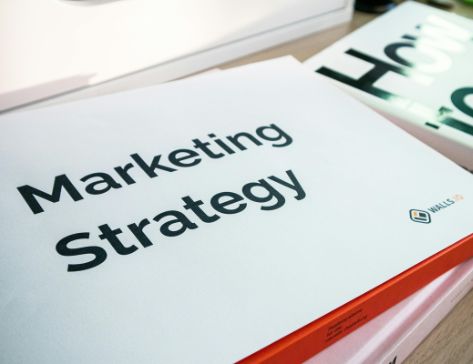
The Evolution of Data-Driven Marketing
The journey from traditional marketing to data science-powered approaches has been transformative. While marketers have always used some form of data, the scope and sophistication of marketing analytics have expanded dramatically in recent years. This evolution has moved through several distinct phases:
- Descriptive Analytics (What happened?): Basic reporting on campaign performance and customer behaviors
- Diagnostic Analytics (Why did it happen?): Identifying factors that influenced marketing outcomes
- Predictive Analytics (What will happen?): Forecasting future customer behaviors and campaign results
- Prescriptive Analytics (What should we do?): Recommending optimal actions based on predicted outcomes
Today’s marketing data scientists operate primarily in the predictive and prescriptive realms, using advanced techniques to anticipate customer needs and optimize marketing strategies before campaigns even launch.
Organizations that integrate data science into their marketing strategy see an average 15-20% improvement in marketing ROI compared to those using traditional approaches, according to recent industry research.
Core Applications of Data Science in Marketing
Data science has transformed virtually every aspect of marketing. Here are the key applications driving results for forward-thinking brands:
1. Customer Segmentation and Persona Development
Data science enables marketers to move beyond basic demographic segmentation to create multidimensional customer profiles based on behaviors, preferences, and predicted future actions. Using clustering algorithms and machine learning techniques, marketers can identify naturally occurring customer segments that would be impossible to discover manually.
2. Customer Journey Analytics
By applying sequential pattern mining and Markov chain models to customer interaction data, data scientists can map the complex paths customers take from awareness to purchase. This analysis reveals critical touchpoints, common friction points, and opportunities to optimize the customer experience.
3. Predictive Lead Scoring
Machine learning models can evaluate leads based on hundreds of variables to predict conversion likelihood, helping sales teams prioritize their efforts on prospects with the highest potential value. These models continuously improve as they incorporate new data about which leads ultimately convert.
4. Content Optimization
Natural language processing and A/B testing frameworks help marketers understand which content resonates with different audience segments. Data science techniques can predict content performance, recommend optimal headlines, and even generate personalized content at scale.
5. Dynamic Pricing Optimization
Pricing algorithms analyze market conditions, competitor behavior, customer willingness to pay, and inventory levels to determine optimal price points that maximize both sales volume and profit margins. These models can adjust prices in real-time based on changing conditions.
6. Attribution Modeling
Advanced attribution models use machine learning to determine the true impact of each marketing touchpoint on conversions, moving beyond simplistic first-touch or last-touch attribution. This enables more accurate assessment of channel performance and more effective budget allocation.
7. Churn Prediction and Prevention
By analyzing patterns in customer behavior, data scientists can identify early warning signs of disengagement and predict which customers are at risk of churning. This enables proactive intervention before customers leave.
Case Study: E-commerce Personalization
An online retailer implemented a data science-driven recommendation engine that analyzed browsing patterns, purchase history, and product attributes to deliver personalized product suggestions. The system used collaborative filtering and deep learning techniques to identify non-obvious relationships between products. Results included:
- 37% increase in average order value
- 24% improvement in conversion rates
- 18% reduction in cart abandonment
Building a Data Science Capability in Marketing: Step-by-Step Guide
Implementing data science in your marketing organization requires a strategic approach:
Step 1: Assess Your Data Readiness
Begin by evaluating your current data ecosystem:
- Inventory available data sources (CRM, web analytics, email, social, etc.)
- Evaluate data quality, completeness, and accessibility
- Identify data gaps that need addressing
- Ensure proper data governance and compliance frameworks
Key deliverable: Data readiness assessment with prioritized improvement plan
Step 2: Define Clear Business Objectives
Identify specific marketing challenges that data science can address:
- Improving customer acquisition efficiency
- Enhancing customer retention and lifetime value
- Optimizing marketing spend allocation
- Personalizing customer experiences at scale
- Accelerating product innovation based on customer insights
Key deliverable: Prioritized list of business use cases with expected impact
Step 3: Build the Right Team
Assemble a cross-functional team with complementary skills:
- Data Scientists: Experts in statistical modeling and machine learning
- Data Engineers: Specialists in data infrastructure and pipeline development
- Marketing Analysts: Professionals who understand marketing metrics and domain context
- Visualization Experts: Team members who can translate insights into accessible dashboards
- Marketing Technologists: Specialists who can integrate insights into marketing platforms
Key deliverable: Staffing plan with roles, responsibilities, and skill requirements
Step 4: Develop Your Technical Infrastructure
Implement the necessary technology stack:
- Data Collection: Tag management, APIs, ETL processes
- Data Storage: Data warehouses, data lakes, customer data platforms
- Analysis Tools: Statistical packages, machine learning frameworks, BI platforms
- Activation Systems: Marketing automation, personalization engines, CRM
Key deliverable: Technical architecture that supports your data science initiatives
Step 5: Start with High-Impact Pilot Projects
Begin with focused initiatives that demonstrate value quickly:
- Select projects with clear business impact and reasonable complexity
- Establish baseline metrics before implementation
- Set clear success criteria and timeline
- Ensure executive sponsorship and stakeholder buy-in
Key deliverable: Pilot project plan with timeline, resources, and success metrics
Step 6: Scale and Operationalize
Expand successful pilots into production systems:
- Develop repeatable processes for model development and deployment
- Create documentation and knowledge sharing mechanisms
- Implement model monitoring and maintenance protocols
- Integrate insights into marketing workflows and decision processes
Key deliverable: Operational framework for scaling data science across marketing
Pro Tip: When building your marketing data science capability, aim for a balance of quick wins and strategic initiatives. Quick wins build momentum and demonstrate value, while strategic projects create sustainable competitive advantage.
Essential Data Science Techniques for Marketers
While marketers don’t need to become data scientists, understanding these key techniques helps them collaborate effectively with technical teams:
Technique | Marketing Application | Business Impact |
---|---|---|
Regression Analysis | Forecasting sales, predicting customer lifetime value | More accurate budget planning and resource allocation |
Classification Algorithms | Lead scoring, churn prediction, sentiment analysis | Improved targeting and proactive customer management |
Clustering | Customer segmentation, market structure analysis | More relevant messaging and product offerings |
Association Rule Mining | Product recommendations, bundle creation | Increased cross-selling and average order value |
Natural Language Processing | Content analysis, social listening, chatbots | Better content strategy and customer service automation |
Time Series Analysis | Seasonal trend detection, demand forecasting | Optimized campaign timing and inventory management |
Overcoming Common Challenges
Organizations often face these obstacles when implementing marketing data science:
Data Silos and Integration Issues
Solution: Implement a customer data platform (CDP) or data lake architecture that unifies information from disparate sources. Establish data governance protocols to ensure consistent data definitions and quality standards.
Skill Gaps and Talent Shortages
Solution: Consider a hybrid approach that combines internal capability building with external partnerships. Invest in training for marketing teams to increase data literacy while leveraging specialized consultants for complex projects.
Balancing Automation and Human Judgment
Solution: Design systems where algorithms augment rather than replace human decision-making. Create feedback loops that allow marketers to provide input on model outputs and continuously improve recommendations.
Privacy Concerns and Regulatory Compliance
Solution: Adopt privacy-by-design principles in all data science initiatives. Implement robust consent management, data minimization practices, and anonymization techniques. Stay current with evolving regulations like GDPR, CCPA, and industry-specific requirements.
Future Trends in Marketing Data Science
The field continues to evolve rapidly. Watch for these emerging developments:
- Automated Machine Learning (AutoML): Tools that automate model selection and hyperparameter tuning, making data science more accessible to marketing teams
- Explainable AI: Models that provide clear rationales for their recommendations, increasing marketer trust and adoption
- Real-time Personalization: Systems that instantly adapt content, offers, and experiences based on current customer context
- Multimodal Analytics: Techniques that combine text, image, voice, and behavioral data for more comprehensive customer understanding
- Federated Learning: Privacy-preserving approaches that build models across decentralized data without compromising sensitive information
- Augmented Analytics: AI-powered systems that automatically generate insights and recommendations from marketing data
Conclusion
Marketing data science represents a fundamental shift in how brands understand and engage with their audiences. By transforming raw data into actionable insights, this discipline enables more precise targeting, personalized experiences, and optimized resource allocation. The result is not just improved marketing performance but a deeper, more valuable relationship with customers based on genuine understanding of their needs and preferences.
The journey to data science maturity in marketing requires investment in people, processes, and technology, but the returns—enhanced customer experiences, increased marketing efficiency, and stronger business results—make it well worth the effort. As consumer expectations continue to rise and competition intensifies, marketing tools powered by data science will become not just a competitive advantage but a fundamental requirement for success.
By following the structured implementation approach outlined in this guide and addressing common challenges proactively, marketers can harness the power of data science to create more effective, efficient, and customer-centric marketing strategies that drive sustainable business growth in the digital age.
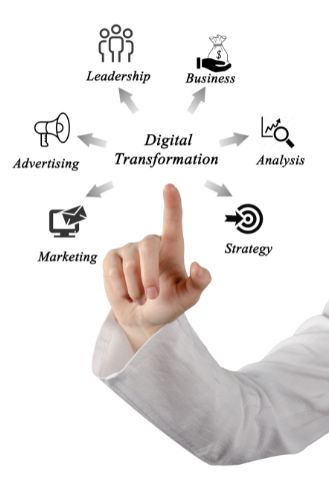