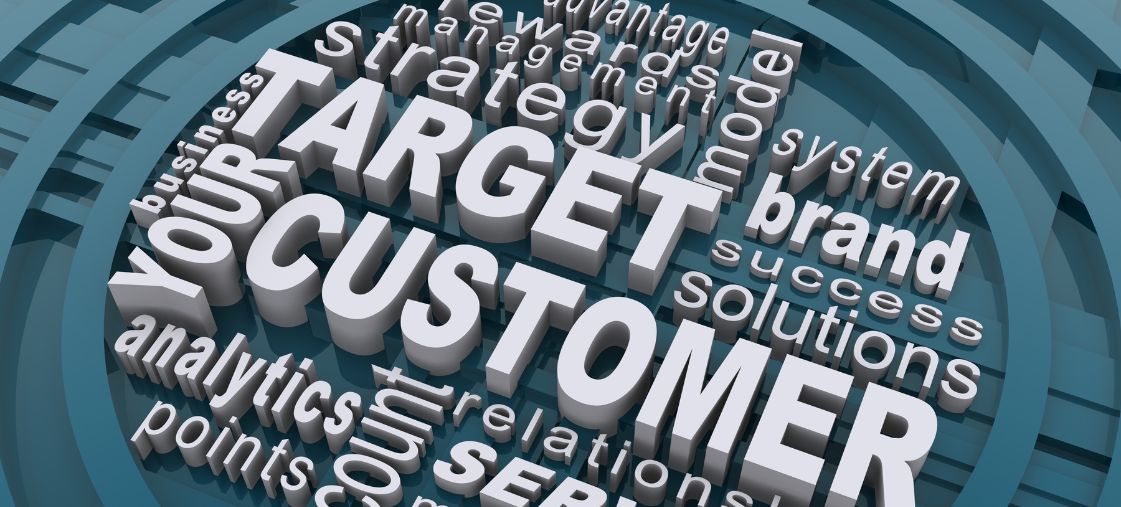
Mastering Customer Intent Analytics: A Data-Driven Approach to Understanding Your Audience
Customer intent analytics has become the cornerstone of effective digital marketing strategies, enabling businesses to decode the underlying motivations that drive consumer behavior across touchpoints. By systematically analyzing signals from search queries, browsing patterns, and interaction data, organizations can gain profound insights into what customers truly want—often before customers themselves fully articulate these desires. This comprehensive guide explores how to leverage analytics to understand customer intent and transform these insights into actionable strategies.
In today’s hyper-competitive digital landscape, simply collecting customer data is insufficient; the real competitive advantage comes from understanding customer intent analysis and applying these insights strategically. Companies that excel at deciphering customer intent can deliver hyper-personalized experiences, optimize marketing spend, and create products and services that genuinely resonate with their target audience’s needs and desires.
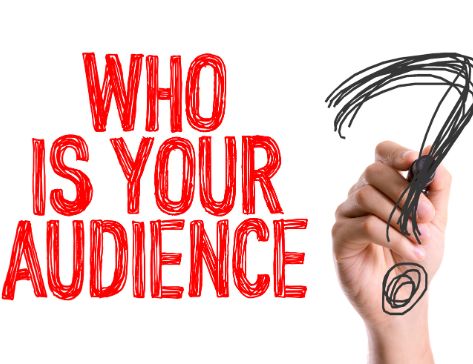
The Four Dimensions of Customer Intent
Before diving into analytics methodologies, it’s essential to understand the primary types of customer intent that your analysis should aim to identify:
1. Informational Intent
When customers seek knowledge, answers, or education about a topic, product, or service. These users are typically in the early stages of the customer journey, gathering information to understand their options.
Example signals: Search queries beginning with “how to,” “what is,” “guide to”; content consumption patterns focused on educational materials; longer time spent on informational pages.
2. Navigational Intent
When customers are looking for a specific website, brand, or destination. These users typically know exactly where they want to go but are using search or navigation as a means to get there.
Example signals: Brand-specific searches; direct URL entries with typos; searches for specific product names or model numbers; navigating directly to specific sections of your site.
3. Commercial Investigation Intent
When customers are researching products or services with the potential to make a purchase but haven’t yet decided. These users are comparing options, reading reviews, and evaluating alternatives.
Example signals: Comparison searches (e.g., “Product A vs. Product B”); review-focused queries; feature-specific searches; multiple product page views; price checking behavior.
4. Transactional Intent
When customers are ready to complete a specific action, such as making a purchase, signing up, or converting. These users have high purchase intent and are looking for the most efficient path to completion.
Example signals: Searches including terms like “buy,” “discount,” “coupon,” “order”; shopping cart interactions; form starts; pricing page visits; high engagement with call-to-action elements.
Building Your Customer Intent Analytics Framework
Step 1: Establish Clear Intent Indicators
Begin by identifying the specific signals that indicate different types of intent within your business context:
- Search Query Analysis: Categorize the keywords and phrases customers use when finding your site
- Behavioral Patterns: Map user flows and interaction patterns that correlate with different intents
- Engagement Metrics: Identify which metrics (time on page, scroll depth, click patterns) align with specific intent types
- Conversion Paths: Analyze the journeys that lead to different conversion types
Create a comprehensive taxonomy of intent signals specific to your business, categorizing them by intent type and assigning relative strength indicators to each signal.
Step 2: Implement Multi-Touchpoint Data Collection
Deploy analytics tools and methodologies that capture intent signals across all customer touchpoints:
- Website Analytics: Configure enhanced event tracking to capture micro-interactions that signal intent
- Search Analytics: Implement both site search tracking and SEO analytics to understand query patterns
- CRM Integration: Connect online behavior with customer profiles and historical interactions
- App Behavior Tracking: Monitor in-app actions and engagement patterns
- Customer Service Interactions: Analyze support tickets, chat logs, and call transcripts for intent signals
- Social Listening: Monitor brand mentions and interactions for intent indicators
The goal is to create a unified data collection framework that captures intent signals regardless of where or how customers interact with your brand.
Step 3: Apply Advanced Analytics Techniques
Leverage sophisticated analytics methodologies to transform raw data into actionable intent insights:
- Machine Learning Classification: Train models to automatically categorize user behavior by intent type
- Natural Language Processing: Analyze text-based interactions (searches, reviews, support tickets) to extract intent
- Sequential Pattern Mining: Identify common sequences of actions that indicate specific intents
- Predictive Intent Modeling: Develop algorithms that forecast likely intent based on early interaction signals
- Cluster Analysis: Group users with similar behavior patterns to identify intent-based segments
- Attribution Modeling: Understand which touchpoints influence intent shifts throughout the customer journey
These advanced techniques allow you to move beyond simple correlation to understand the causal relationships between behaviors and underlying intents.
Step 4: Create Intent-Based Customer Segments
Develop dynamic customer segments based on identified intent patterns:
- Primary Intent Segments: Group customers by their dominant intent type
- Intent Progression Segments: Categorize users based on their movement between intent types
- Intent Intensity Segments: Differentiate between casual browsers and highly motivated searchers
- Multi-Intent Segments: Identify users exhibiting multiple simultaneous intent types
- Intent Volatility Segments: Group users based on how frequently their intent shifts
These intent-based segments provide a more actionable foundation for personalization than traditional demographic or behavioral segments alone.
Step 5: Implement Intent-Responsive Strategies
Develop systematic approaches to respond to different customer intents:
Intent Type | Strategic Response | Implementation Tactics |
---|---|---|
Informational | Educational Content Delivery | – Surface relevant guides and tutorials – Offer interactive learning tools – Provide downloadable resources – Suggest related informational content |
Navigational | Streamlined Path Provision | – Simplify navigation to desired destinations – Offer direct shortcuts to common destinations – Implement intelligent search suggestions – Create personalized navigation elements |
Commercial | Evaluation Facilitation | – Present side-by-side comparisons – Highlight differentiating features – Surface relevant reviews and testimonials – Offer interactive product explorers |
Transactional | Conversion Optimization | – Streamline checkout processes – Present relevant offers and incentives – Remove friction points – Provide reassurance elements |
The key is developing systematic, scalable responses to each intent type that can be automatically triggered based on detected signals.
Advanced Intent Analytics Implementation Techniques
Real-Time Intent Detection
Implement systems that identify and respond to intent signals as they occur:
- Deploy edge computing solutions that process intent signals with minimal latency
- Implement streaming analytics that continuously evaluate user behavior patterns
- Create dynamic content systems that adapt in real-time to detected intent shifts
- Develop intent-based triggering rules for marketing automation platforms
Intent Journey Mapping
Visualize and analyze how customer intent evolves throughout the relationship lifecycle:
- Create intent-focused journey maps that track intent shifts across touchpoints
- Identify common intent progression patterns and their correlation with conversion
- Analyze intent “dead ends” where users fail to progress to the next logical intent stage
- Develop intent nurturing strategies that guide users through appropriate intent sequences
Cross-Channel Intent Consistency
Ensure that detected intent informs interactions across all channels:
- Implement unified customer profiles that include intent data accessible across touchpoints
- Create intent handoff protocols that maintain context when users switch channels
- Develop channel-specific intent response strategies that maintain consistent objectives
- Measure cross-channel intent alignment and identify disconnects
Intent-Based Personalization at Scale
Move beyond basic personalization to intent-driven experiences:
- Implement dynamic content systems that adapt based on detected intent signals
- Create intent-specific messaging hierarchies that prioritize relevant information
- Develop adaptive user interfaces that reorganize based on likely user goals
- Build recommendation engines that factor in current intent alongside historical preferences
Measuring Intent Analytics Success
Establish comprehensive metrics to evaluate the effectiveness of your intent analytics program:
- Intent Classification Accuracy: Measure how accurately your systems identify true user intent
- Intent-to-Conversion Correlation: Track how different intent types and patterns relate to conversion outcomes
- Intent Fulfillment Rate: Assess how effectively your site/app satisfies the identified intent
- Intent Shift Influence: Measure your ability to guide users from early-stage to conversion-stage intent
- Intent-Based Personalization Lift: Compare performance of intent-based vs. traditional personalization
- Cross-Channel Intent Consistency: Evaluate how seamlessly intent context transfers between channels
Common Intent Analytics Challenges and Solutions
Challenge: Ambiguous Intent Signals
Solution: Implement confidence scoring for intent classification, using multiple signal types to confirm intent, and developing fallback strategies for low-confidence scenarios.
Challenge: Rapidly Shifting Intent
Solution: Deploy real-time analytics systems, create intent volatility handling protocols, and design experiences that accommodate multiple simultaneous intents.
Challenge: Privacy Constraints
Solution: Focus on contextual and session-based intent signals, implement privacy-by-design principles, and create transparent value exchanges for intent data sharing.
Challenge: Organizational Silos
Solution: Establish cross-functional intent teams, create shared intent taxonomies and metrics, and implement unified intent data platforms accessible across departments.
Conclusion
Mastering customer intent analytics represents one of the most significant opportunities for organizations to gain competitive advantage in the digital economy. By systematically collecting, analyzing, and responding to intent signals, businesses can create experiences that feel remarkably intuitive and personalized from the customer perspective.
The most successful organizations will move beyond basic intent recognition to develop sophisticated systems that can detect nuanced intent patterns, predict intent shifts before they occur, and seamlessly adapt experiences across channels. By implementing the right analytics tools and methodologies, companies can transform their approach to customer understanding, creating experiences that genuinely resonate with what customers are trying to accomplish at each moment of interaction.
Key Takeaway: Customer intent analytics is not merely a technical implementation but a fundamental shift in perspective—moving from asking “what are customers doing?” to understanding “what are customers trying to accomplish?” This intent-focused mindset, supported by robust analytics capabilities, forms the foundation of truly customer-centric digital experiences.
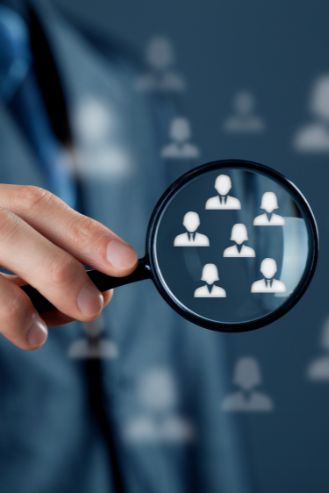