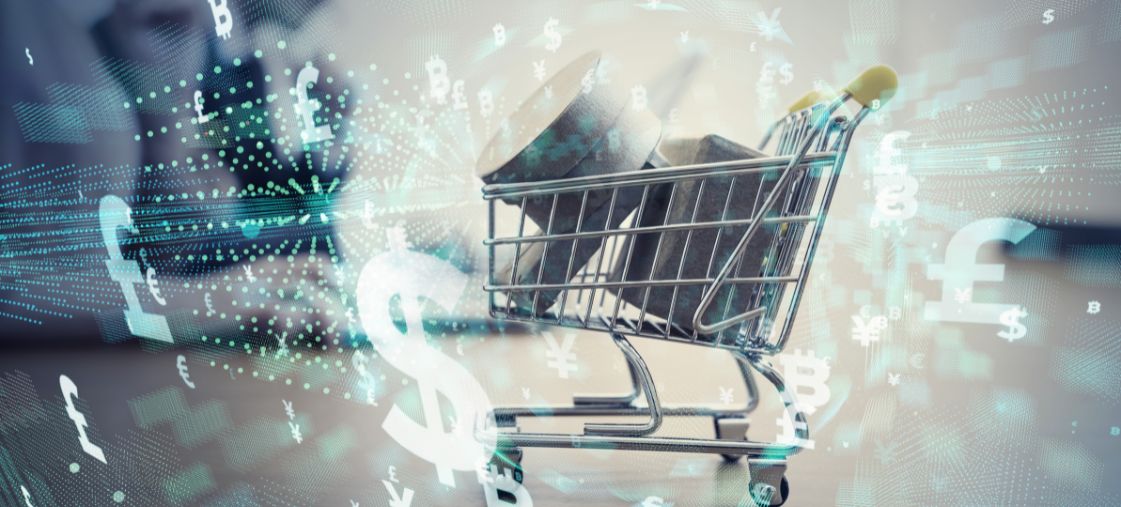
How E-commerce Predictive Analytics Can Transform Your Online Business in 2025
E-commerce predictive analytics has emerged as a game-changing technology that enables online retailers to anticipate customer behavior, optimize inventory, personalize shopping experiences, and make data-driven decisions that significantly impact their bottom line. In today’s hyper-competitive online retail landscape, businesses that leverage predictive analytics gain a substantial competitive advantage by moving from reactive to proactive strategies, allowing them to stay ahead of market trends and customer expectations.
The exponential growth of online shopping has generated vast amounts of customer data, creating both challenges and opportunities for e-commerce businesses. According to recent industry reports, companies implementing predictive analytics in e-commerce experience an average 15-20% increase in conversion rates and a 25% reduction in customer acquisition costs. As digital commerce continues to evolve, predictive analytics has transitioned from a luxury to a necessity for businesses seeking sustainable growth in increasingly crowded marketplaces.
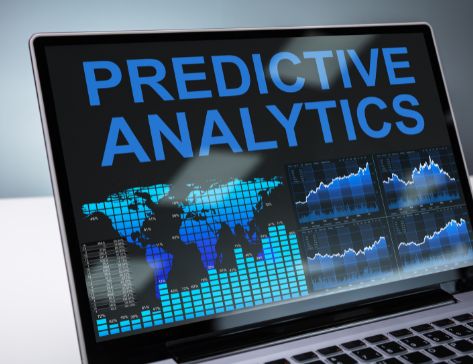
Understanding E-commerce Predictive Analytics
Predictive analytics in e-commerce refers to the use of historical data, statistical algorithms, and machine learning techniques to identify patterns and predict future outcomes and trends. Unlike traditional analytics that provides insights into what has happened, predictive analytics forecasts what will happen, enabling proactive decision-making.
The core components of e-commerce predictive analytics include:
- Data Collection: Gathering relevant customer, product, and transaction data from multiple sources
- Data Processing: Cleaning, normalizing, and preparing data for analysis
- Predictive Modeling: Applying statistical models and machine learning algorithms to identify patterns and make predictions
- Visualization and Reporting: Presenting insights in accessible formats for decision-makers
- Implementation: Integrating predictions into business processes and customer experiences
- Continuous Improvement: Refining models based on new data and outcomes
Key Distinction: While descriptive analytics tells you what happened and diagnostic analytics explains why it happened, predictive analytics forecasts what will happen, and prescriptive analytics suggests what actions to take based on those predictions.
Key Applications of Predictive Analytics in E-commerce
1. Customer Behavior Prediction
Anticipate how customers will interact with your store:
- Purchase Propensity Modeling: Identify which customers are most likely to make a purchase in the near future
- Churn Prediction: Detect customers at risk of abandoning your brand
- Lifetime Value Forecasting: Estimate the long-term value of customer relationships
- Browse-to-Buy Conversion: Predict which browsing behaviors are most likely to lead to purchases
- Next Purchase Prediction: Forecast what products a customer is likely to buy next
Implementation Example: An online fashion retailer used purchase propensity models to identify customers with a high likelihood of purchasing seasonal items, resulting in a 35% increase in campaign conversion rates and a 20% reduction in marketing costs.
2. Dynamic Pricing Optimization
Set optimal prices based on multiple factors:
- Demand Forecasting: Predict how demand will fluctuate based on various factors
- Competitive Price Analysis: Automatically adjust prices in response to competitor changes
- Price Elasticity Modeling: Understand how price changes affect demand for specific products
- Personalized Pricing: Offer individualized discounts based on customer value and behavior
- Promotion Effectiveness Prediction: Forecast the impact of different promotional strategies
Implementation Example: An electronics e-commerce site implemented dynamic pricing algorithms that adjusted prices based on demand forecasts, competitor pricing, and inventory levels, resulting in a 15% increase in profit margins while maintaining competitive market positioning.
3. Inventory and Supply Chain Optimization
Manage inventory more efficiently with advanced marketing data infrastructure and predictive tools:
- Demand Forecasting: Predict product demand across different timeframes and regions
- Stock Level Optimization: Maintain optimal inventory levels to balance costs and availability
- Reorder Point Prediction: Automatically determine when to restock products
- Seasonal Trend Analysis: Anticipate seasonal fluctuations in demand
- Supply Chain Disruption Prediction: Identify potential issues before they impact operations
Implementation Example: A home goods e-commerce company reduced inventory costs by 23% while decreasing stockouts by 45% by implementing predictive demand forecasting that accounted for seasonal trends, marketing activities, and external factors like weather patterns.
4. Personalized Product Recommendations
Deliver tailored product suggestions to each customer:
- Collaborative Filtering: Recommend products based on similar customers’ preferences
- Content-Based Filtering: Suggest items similar to those a customer has shown interest in
- Hybrid Recommendation Systems: Combine multiple approaches for more accurate suggestions
- Real-Time Personalization: Adjust recommendations based on current browsing behavior
- Cross-Sell and Upsell Prediction: Identify complementary or premium products to suggest
Implementation Example: An online bookstore implemented a hybrid recommendation engine that increased average order value by 28% and improved customer engagement metrics, with users spending 40% more time on the site exploring recommended titles.
5. Customer Segmentation and Targeting
Create dynamic customer segments for targeted marketing:
- Behavioral Segmentation: Group customers based on their actions and engagement patterns
- Value-Based Segmentation: Categorize customers by their current and potential value
- Needs-Based Clustering: Identify groups with similar product needs and preferences
- Dynamic Micro-Segmentation: Create and update highly specific customer segments in real-time
- Lookalike Audience Modeling: Find new customers who resemble your best existing customers
Implementation Example: A health and wellness e-commerce site used predictive segmentation to create dynamic customer groups based on purchase history, browsing behavior, and engagement patterns, resulting in a 32% increase in email campaign performance and a 24% improvement in retargeting ad efficiency.
Implementing Predictive Analytics in Your E-commerce Business
Follow this step-by-step framework to successfully implement predictive analytics in your online store:
Step 1: Define Clear Business Objectives
Begin by identifying specific business challenges that predictive analytics can address:
- Prioritize use cases based on potential business impact and implementation feasibility
- Set measurable goals and KPIs for each predictive analytics initiative
- Ensure alignment between analytics objectives and overall business strategy
- Start with 1-2 high-impact use cases rather than attempting a comprehensive implementation
- Define success criteria and expected ROI for each project
Pro Tip: Create a prioritization matrix that scores potential predictive analytics use cases based on business impact, technical feasibility, data availability, and implementation time to identify the most promising starting points.
Step 2: Assess Data Readiness and Infrastructure
Evaluate your current data ecosystem and identify gaps:
- Inventory available data sources (customer data, transaction history, product data, etc.)
- Assess data quality, completeness, and accessibility
- Identify data collection gaps that need to be addressed
- Evaluate your current analytics infrastructure and capabilities
- Determine whether you need to build, buy, or partner for predictive analytics capabilities
Pro Tip: Conduct a data quality audit focusing on the specific data elements needed for your priority use cases, rather than attempting to address all data quality issues simultaneously.
Step 3: Select the Right Tools and Technologies
Choose appropriate solutions based on your needs and capabilities:
- Evaluate predictive analytics platforms specifically designed for e-commerce
- Consider cloud-based solutions that offer scalability and reduced infrastructure requirements
- Assess build vs. buy options based on your team’s technical capabilities
- Ensure selected tools integrate with your existing e-commerce platform and data sources
- Consider future scalability needs as your predictive analytics capabilities mature
Pro Tip: For smaller e-commerce businesses, start with predictive analytics features built into your existing platforms (e.g., Shopify, Magento) before investing in standalone solutions.
Step 4: Develop and Deploy Predictive Models
Create and implement models tailored to your specific use cases:
- Prepare and preprocess data for modeling (cleaning, normalization, feature engineering)
- Select appropriate algorithms based on your specific prediction needs
- Train models using historical data and validate performance
- Implement models in a test environment before full deployment
- Develop processes for model monitoring and performance evaluation
Pro Tip: Start with simpler models that are easier to interpret and implement before moving to more complex approaches. Often, a well-designed simple model can deliver significant value while being easier to maintain and explain to stakeholders.
Step 5: Integrate Predictions into Business Processes
Ensure predictive insights drive actual business actions:
- Develop automated workflows that translate predictions into actions
- Integrate predictive outputs with customer-facing systems (website, email, apps)
- Create dashboards and reporting tools for business users
- Train staff on how to interpret and act on predictive insights
- Establish feedback loops to capture the results of prediction-driven actions
Pro Tip: Create “prediction-to-action” playbooks that clearly define what specific actions should be taken based on different predictive insights, ensuring that predictions consistently translate to business value.
Step 6: Measure, Learn, and Iterate
Continuously improve your predictive analytics capabilities:
- Implement A/B testing to measure the impact of prediction-driven actions
- Monitor model performance and accuracy over time
- Retrain models regularly with new data
- Gather feedback from business users on the utility of predictions
- Expand to additional use cases as you build capabilities and demonstrate value
Pro Tip: Establish a regular cadence for model evaluation and retraining, with more frequent updates for fast-changing aspects of your business (like pricing) and less frequent updates for more stable predictions (like seasonal trends).
Common Challenges and Solutions
Challenge: Data Quality and Integration Issues
Solution: Implement data quality monitoring tools, establish data governance practices, and consider a customer data platform (CDP) to unify data from multiple sources. Focus on improving data quality for specific high-value use cases rather than trying to fix all data issues simultaneously.
Challenge: Lack of Technical Expertise
Solution: Consider e-commerce-specific predictive analytics solutions with user-friendly interfaces, partner with analytics service providers, invest in training for key team members, or hire specialized talent. Many modern platforms offer “predictive analytics as a service” with minimal technical requirements.
Challenge: Balancing Personalization and Privacy
Solution: Implement transparent data practices, obtain proper consent for data usage, anonymize data where possible, and ensure compliance with regulations like GDPR and CCPA. Focus on delivering value through personalization that customers appreciate rather than creating experiences that feel invasive.
Challenge: Measuring ROI of Predictive Analytics
Solution: Establish clear baseline metrics before implementation, use controlled experiments to isolate the impact of predictive features, track both direct metrics (conversion, AOV) and indirect benefits (customer satisfaction, retention), and create dashboards that connect predictive initiatives to business outcomes.
Case Studies: E-commerce Predictive Analytics Success Stories
Company Type | Challenge | Predictive Solution | Results |
---|---|---|---|
Fashion Retailer | High inventory costs and frequent stockouts | Demand forecasting and inventory optimization | 30% reduction in inventory costs, 40% decrease in stockouts |
Electronics Store | Low email marketing engagement | Personalized product recommendations and send-time optimization | 65% increase in email click-through rates, 28% higher conversion |
Beauty Products | High customer acquisition costs | Lookalike audience modeling and churn prediction | 35% reduction in CAC, 25% improvement in retention |
Home Goods | Suboptimal pricing strategy | Dynamic pricing and elasticity modeling | 18% increase in profit margins without significant volume loss |
Subscription Box | High churn rate | Early churn prediction and proactive intervention | 40% reduction in voluntary churn, 22% increase in LTV |
Future Trends in E-commerce Predictive Analytics
Stay ahead of the curve by monitoring these emerging developments:
- AI-Powered Visual Search: Predictive systems that understand and recommend products based on images
- Voice Commerce Prediction: Anticipating customer needs in voice-based shopping environments
- Emotion AI: Detecting and responding to customer emotional states during the shopping journey
- Hyper-Personalization: Moving beyond segments to true one-to-one personalized experiences
- Predictive Returns Management: Identifying likely returns before they happen and taking preventive action
- Augmented Analytics: AI-assisted insights generation that makes predictive capabilities accessible to non-technical users
- Federated Learning: Privacy-preserving predictive techniques that don’t require centralized data storage
Conclusion
E-commerce predictive analytics represents a transformative approach to online retail, enabling businesses to anticipate customer needs, optimize operations, and deliver personalized experiences at scale. By systematically implementing predictive capabilities across key business functions, e-commerce companies can achieve significant competitive advantages in customer acquisition, conversion, retention, and lifetime value.
The journey to predictive analytics maturity requires thoughtful planning, appropriate technology selection, and a commitment to data-driven decision making. However, the potential returns—in the form of increased revenue, improved efficiency, and enhanced customer experiences—make it a worthwhile investment for e-commerce businesses of all sizes. As online competition continues to intensify, predictive analytics will increasingly separate market leaders from followers, making it an essential capability for e-commerce businesses seeking sustainable growth in the digital marketplace.
Key Takeaway: E-commerce predictive analytics is no longer a competitive advantage but a competitive necessity. By starting with high-impact use cases, ensuring data readiness, selecting appropriate tools, and integrating predictions into business processes, online retailers can harness the power of predictive analytics to drive measurable business results and create superior customer experiences.
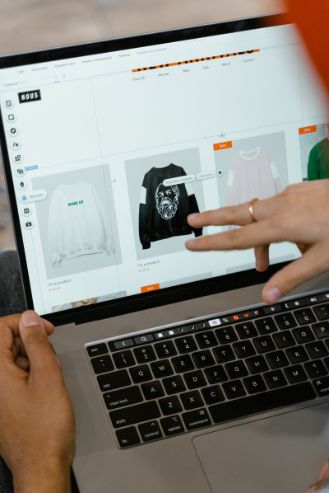