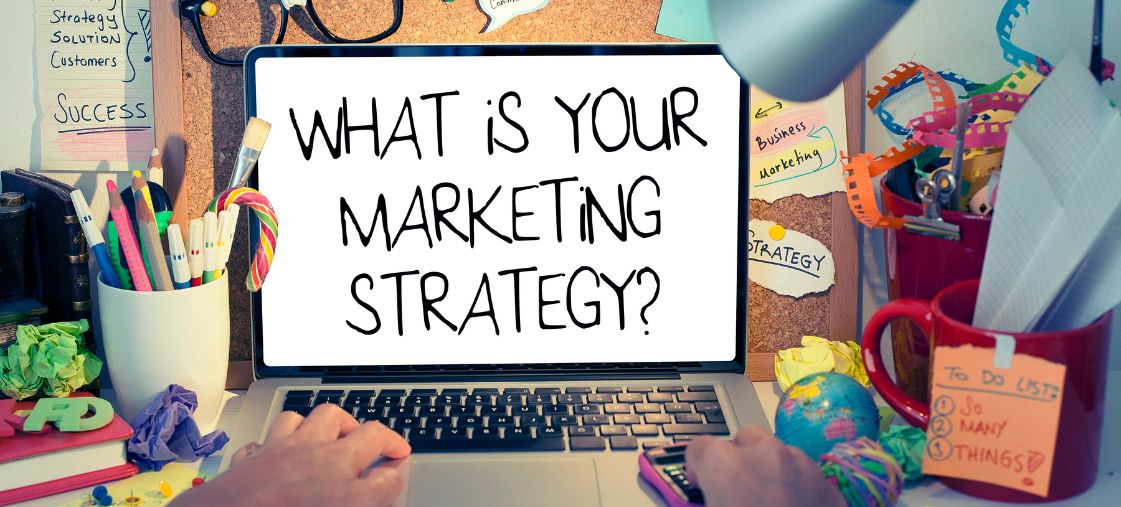
Machine Learning in Marketing: A Beginner's Guide to Transforming Your Strategy
This beginner’s guide explores the fundamentals of Machine Learning in Marketing, demystifying how this powerful subset of Artificial Intelligence (AI) is revolutionizing the way businesses connect with customers. If terms like “algorithms,” “neural networks,” and “big data” seem daunting, fear not. At its core, machine learning (ML) empowers computer systems to learn from data, identify patterns, and make decisions or predictions with minimal human intervention. For marketers, this translates into unprecedented capabilities for personalization, prediction, and optimization, moving beyond traditional methods towards truly intelligent strategies.
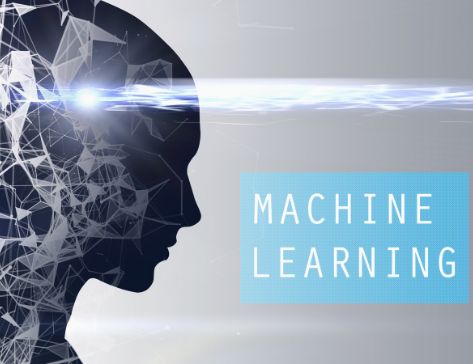
What Exactly is Machine Learning (Simplified)?
Think of machine learning like teaching a computer by showing it lots of examples, rather than programming it with explicit rules for every possible scenario. Instead of telling a program “If a customer buys product A and product B, then show them product C,” you feed an ML model vast amounts of historical customer data (purchases, browsing history, demographics, interactions). The model then learns the underlying patterns and associations itself. It might discover complex relationships you wouldn’t have thought to program, ultimately figuring out *on its own* which customers are most likely to be interested in product C, or D, or E, based on their unique behavioral footprint. There are different ways machines learn, such as being guided with labeled examples (supervised learning) or finding patterns in unlabeled data (unsupervised learning), concepts often explored in resources like this beginner guide to machine learning found on community forums.
Why is Machine Learning a Game-Changer for Marketing?
Traditional marketing often relies on broad segments, manual analysis of past performance, and educated guesses. Machine learning elevates this significantly:
- Handling Data Overload: Humans simply cannot process the sheer volume and complexity of data generated by modern customer interactions across multiple channels. ML algorithms thrive on this data, uncovering insights hidden within.
- True Personalization at Scale: ML enables marketers to move beyond basic segmentation (e.g., age, location) to hyper-personalization based on individual behaviors, preferences, and predicted future actions, delivering unique experiences to millions of customers simultaneously.
- Predictive Power: Instead of just reporting what happened, ML models can forecast future outcomes – predicting which customers might churn, which leads will convert, or how much revenue a campaign might generate.
- Automation and Efficiency: ML can automate repetitive tasks like ad bidding, email send time optimization, and content recommendations, freeing up marketing teams to focus on strategy and creativity.
- Continuous Improvement: ML models learn and adapt over time as new data comes in, constantly refining their performance and ensuring marketing efforts stay relevant.
Key Applications of Machine Learning in Marketing
The practical applications of ML in marketing are vast and growing. Here are some of the most impactful areas where it’s making a difference, highlighting the urgency for marketing teams to adopt these capabilities:
1. Advanced Customer Segmentation
Instead of manually defining segments based on demographics, ML (specifically unsupervised learning techniques like clustering) can analyze customer data to identify natural groupings based on subtle behavioral patterns, purchase history, engagement levels, or predicted lifetime value. This reveals hidden, high-value micro-segments that traditional methods would miss.
2. Predictive Analytics (Lead Scoring & Churn Prediction)
This is a major application area. ML models (using supervised learning) can analyze historical data to predict future events.
- Predictive Lead Scoring: Assigns a score to leads based on their likelihood to convert, allowing sales teams to prioritize efforts effectively.
- Churn Prediction: Identifies customers at high risk of leaving, enabling proactive retention campaigns before it’s too late.
- Customer Lifetime Value (CLV) Prediction: Forecasts the total revenue a business can expect from a customer over their entire relationship.
3. Personalization and Recommendation Engines
Perhaps the most visible application. ML powers the recommendation engines used by Netflix, Amazon, Spotify, and countless e-commerce sites. By analyzing past behavior (views, purchases, ratings) of individual users and similar users, ML algorithms predict what content, products, or services a user is most likely to engage with next, delivering highly personalized experiences.
4. Sentiment Analysis
Using Natural Language Processing (NLP), a branch of ML, algorithms can analyze text data (customer reviews, social media comments, survey responses, support tickets) to determine the underlying sentiment – positive, negative, or neutral. This helps marketers understand brand perception, identify customer pain points, and track reactions to campaigns in real-time.
5. Ad Campaign Optimization and Programmatic Bidding
ML algorithms optimize digital advertising spend in real-time. They analyze vast amounts of data (user behavior, ad placement, time of day, device type) to predict which ad impressions are most likely to lead to conversions. This powers programmatic advertising platforms, automating bidding strategies to maximize ROI and reach the right audience at the right moment.
6. Dynamic Pricing
ML models can analyze demand, competitor pricing, inventory levels, customer purchase history, and perceived willingness to pay to set optimal prices dynamically. This is common in industries like travel and e-commerce, helping businesses maximize revenue and manage inventory effectively.
7. Intelligent Chatbots and Customer Service
ML-powered chatbots (using NLP) can understand customer queries, provide instant answers to common questions, guide users through processes, and even handle simple transactions 24/7. This improves customer experience and frees up human agents for more complex issues.
Getting Started: Tips for Marketing Beginners
Embarking on the ML journey doesn’t necessarily require a PhD in computer science. Here’s how marketing teams can begin:
- Define Clear Marketing Goals: What specific problem do you want ML to solve? Increase conversion rates? Reduce churn? Improve personalization? Start with a clear objective.
- Focus on Data Quality: ML models need good data. Ensure your data collection is accurate, consistent, and relevant to your goals. Address data silos where possible.
- Leverage Built-in ML Features: Many modern marketing platforms (CRMs, marketing automation tools, email service providers, ad platforms) now incorporate ML features (e.g., predictive lead scoring, send time optimization, automated segmentation). Start by exploring and utilizing these existing capabilities.
- Explore User-Friendly ML Platforms: Cloud providers (Google Cloud AI, AWS SageMaker, Azure Machine Learning) offer platforms with varying levels of complexity, including some with automated ML (AutoML) features that simplify model building.
- Educate Yourself (or Collaborate): Understand the basic concepts of ML and its marketing applications. Numerous online courses and resources are available. Alternatively, collaborate with data scientists or analysts within your organization or consider external consultants for specific projects.
- Start Small and Iterate: Don’t try to implement everything at once. Pick one specific use case, run a pilot project, measure the results, learn, and then scale or try another application.
Challenges to Keep in Mind
While powerful, implementing ML in marketing involves considerations:
- Data Privacy and Ethics: Handling customer data requires strict adherence to privacy regulations (GDPR, CCPA) and ethical guidelines. Transparency is key.
- Data Requirements: ML models often require large amounts of high-quality data to perform well.
- Complexity and Expertise: Building custom ML models can require specialized skills, although AutoML tools are lowering the barrier.
- Potential for Bias: If the training data reflects historical biases, the ML model may perpetuate or amplify them. Careful monitoring and mitigation strategies are needed.
- Integration: Integrating ML insights seamlessly into existing marketing workflows and tools can be challenging.
“Machine learning is the science of getting computers to act without being explicitly programmed.” – Arthur Samuel. In marketing, it’s about enabling systems to understand and anticipate customer needs intelligently.
Conclusion: Embracing the Intelligent Future of Marketing
Machine Learning in Marketing is not just hype; it’s a fundamental shift towards more intelligent, efficient, and customer-centric strategies. By enabling computers to learn from data, marketers can unlock deeper insights, deliver hyper-personalized experiences, predict future behavior, and optimize campaigns with unprecedented accuracy. While challenges exist, the increasing accessibility of ML tools and platforms means that businesses of all sizes can start leveraging its power. From refining customer segmentation and predicting churn to optimizing ad spend on platforms displaying diverse content like native advertising, ML offers a transformative toolkit. For marketing teams looking to stay competitive and drive meaningful results, understanding and embracing machine learning is no longer optional – it’s the path forward.
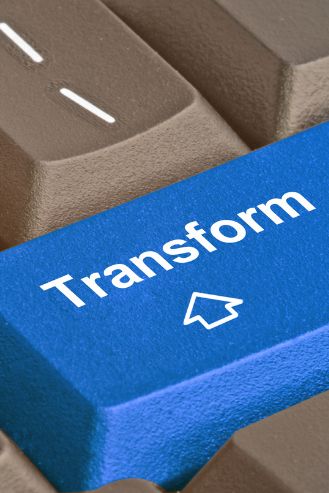